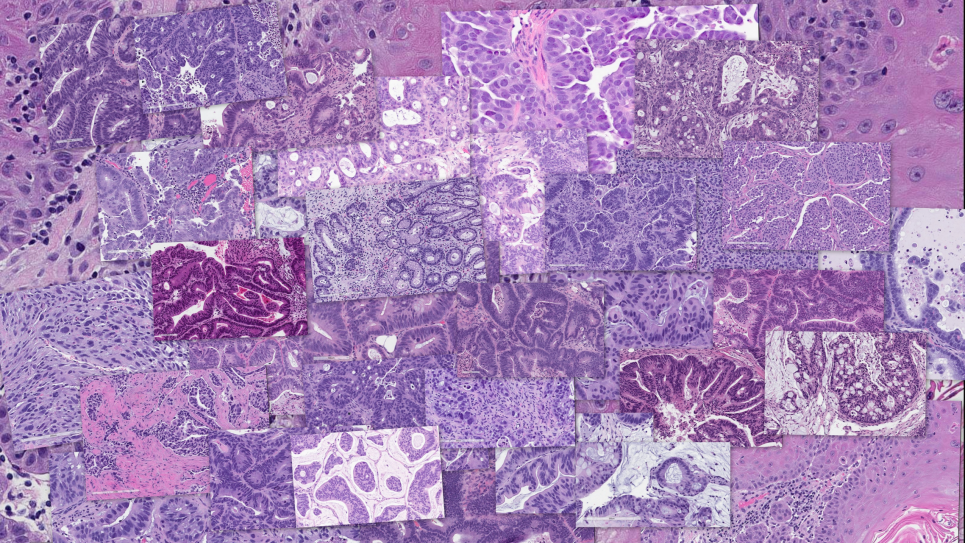
Predicting cancer type and drug response using histopathology images from the National Cancer Institute’s Patient-Derived Models Repository. Image: Rick Stevens, Argonne National Laboratory
This project attacks the cancer “drug response problem” using a deep learning workflow that will search billions of virtual drug combinations aided by predictive models of cellular drug response.
Utilizing data frames too large for conventional systems and a deep learning workflow designed to provide new approaches to personalized cancer medicine, this project, aiming to dramatically decrease the time necessary for successful drug development, enables billions of virtual drugs to be screened singly and in numerous combinations, while predicting their effects on tumor cells. The workflow is built from the CANDLE (CANcer Distributed Learning Environment) framework to optimize model hyper-parameters and perform billions of inferences to quantify model uncertainty and ultimately deliver results to be tested in pre-clinical experiments.