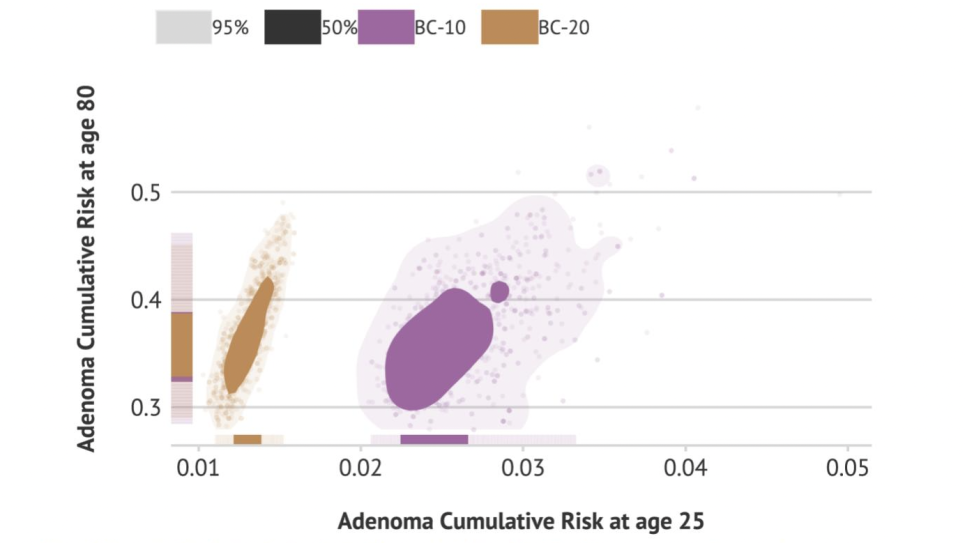
Posterior distribution for Cumulative Adenoma initiation Risk. Shaded areas represent the posterior distribution of cumulative adenoma initiation risk for each model. Shaded areas correspond to 50% and 95% highest density regions. A 95% Highest-density interval summarizes a distribution as an interval that spans 95% of it, such that all points within the interval have higher probability than any point outside the interval. Shaded areas close to axes reflect marginal highest density regions, which do not need to match joint in- terval. Colors represent the two models, BC-10 and BC-20.