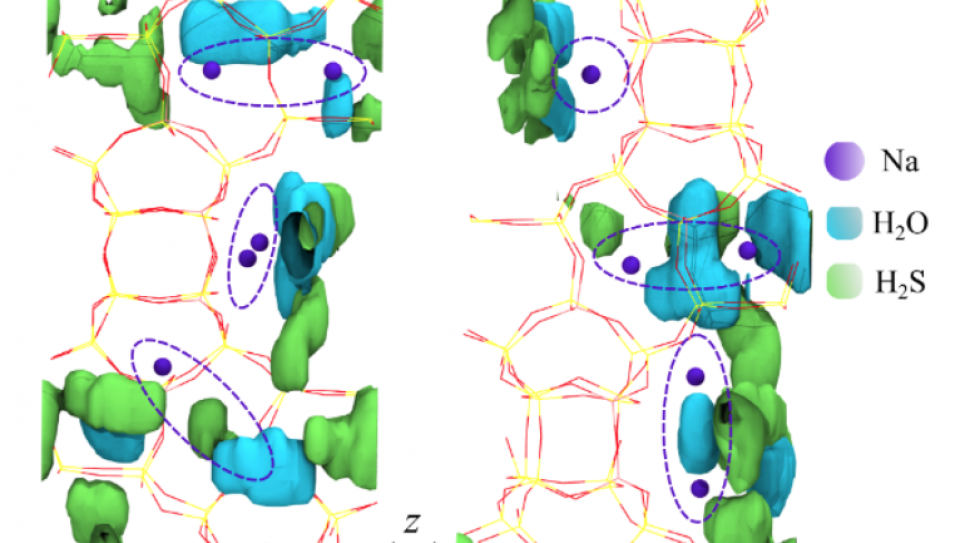
Functional Nanoporous Materials. Image: J. Ilja Siepmann
An interdisciplinary, collaborative team will use predictive hierarchical modeling and machine learning to accelerate the discovery and design of materials for a variety of energy-related applications and to advance scientific and technological capabilities with innovative discoveries.
An interdisciplinary, collaborative team will use predictive hierarchical modeling and machine learning to accelerate the discovery and design of materials for a variety of energy-related applications and to advance scientific and technological capabilities with innovative discoveries. The research objectives of this ALCC proposal are aligned with the goals of the DOE-funded Nanoporous Materials Genome Center (NMGC). Research focus is on four topics:
Work enabled by prior ALCC allocations has led to the award of one US patent for the ethanol purification.