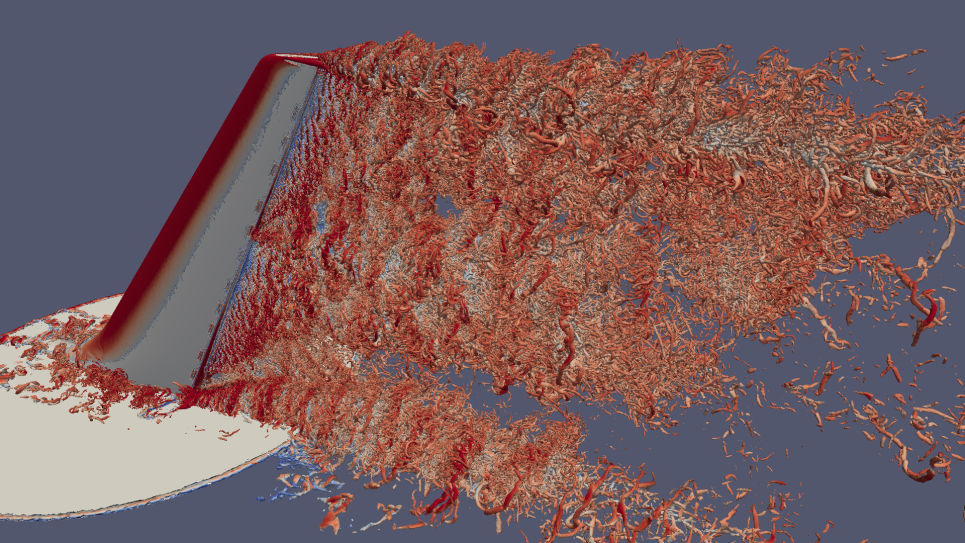
Isosurface of instantaneous Q criterion colored by speed over a vertical tail at Re = 3.5 105, with a rudder deflection angle of 30◦and a single unsteady jet active (fifth from the top). This DDES simulation on a 5 billion element mesh, run on 64k KNL cores on Theta, shows our ability to refine the grid to capture the unsteady structures resulting from the separation near the rudder and from the interaction between the unsteady jet and the crossflow.