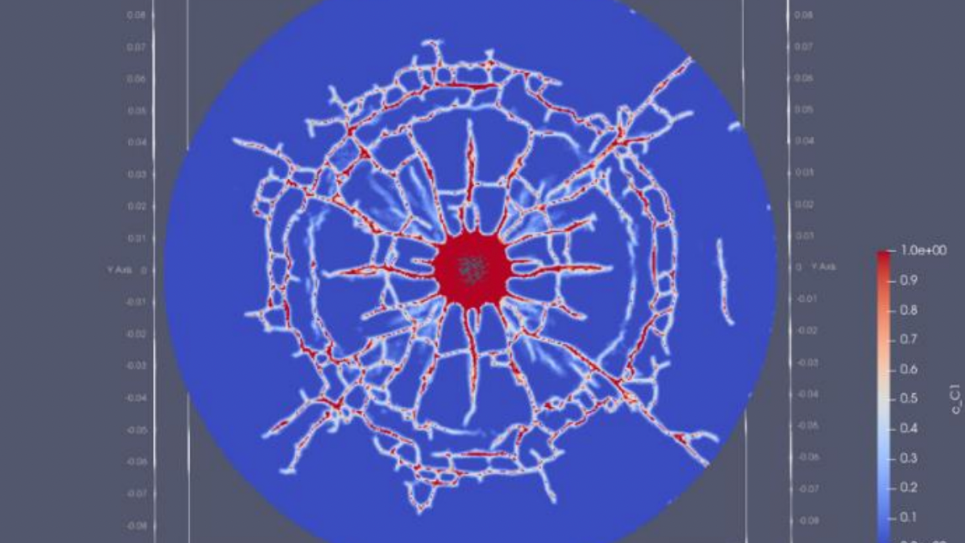
Damage pattern of disk, with blue color indicating zero percent broken bonds and red color represents 100% broken bonds.
The main objective of this research project is to develop a data-driven operator level surrogate model (DeepONet) to take the place of atomic scale modeling in a time-dependent multiscale system. As noted by DeepMind researcher Irina Higgins, “Once DeepONet is trained, it can be applied to new input functions, thus producing new results substantially faster than numerical solvers.”
The research work is inspired by the increasing preference for data-driven models for creating atomisticcontinuum multi-scale fracture analysis approaches employing machine learning techniques. Understanding fracture mechanics requires modeling of deformation and crack growth in material microstructures. The complex mechanisms involved in the process of crack growth operate on a variety of spatial scales, from the atomic scale of fracture initiation to the continuum scales of short crack propagation along with the microstructure deformation. Pure atomistic simulations using molecular dynamics (MD) can be used to investigate these processes. It is still very difficult to perform large-scale MD simulations of fracture propagation in larger domains that correspond to continuum length and time scales. Furthermore, the computationally demanding high-fidelity numerical solvers that can precisely anticipate the relevant parameters demand a fine-grained fracture resolution. Besides that, independent, expensive simulations must be run for every little change in the domain parameters and/or material properties. Partially getting around this restriction requires combining the continuum and atomistic models. The remaining portions of the domain are represented using continuum-scale constitutive relations, while critical sections with high stress intensity (crack tip) are modeled using MD at the atomic scale.
This project will be the first of its kind to provide a unified fracture analysis model that can be used for failure analysis in any engineering material. It will also be a one-shot solution to determine the likely fracture path in order to prevent unexpected failures. By organizing materials in unique ways, based on predetermined failure paths, this research opens up the possibility of synthesizing a material with customized structural properties and the optimal topology and shape. It is directly related to DOE mission since this is a part of the PhILMs collaboratory is directing.