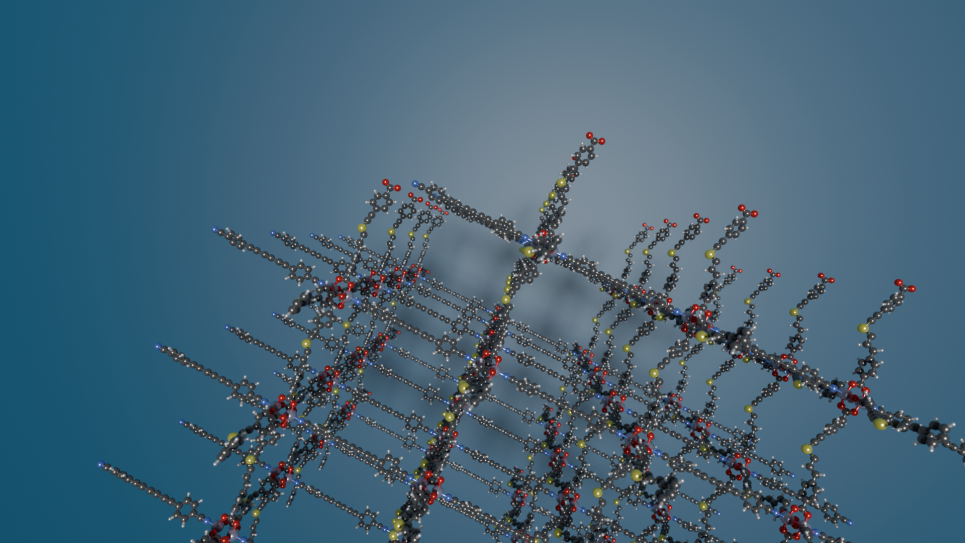
A high-performance metal-organic framework generated by MOFA in a 450-node computing run on the ALCF’s Polaris system. Atoms shown include carbon (brown), oxygen (red), hydrogen (white), sulfur (yellow), zinc (large grey), and nitrogen (small blue/white). Image: Xiaoli Yan, Argonne National Laboratory and University of Illinois Chicago