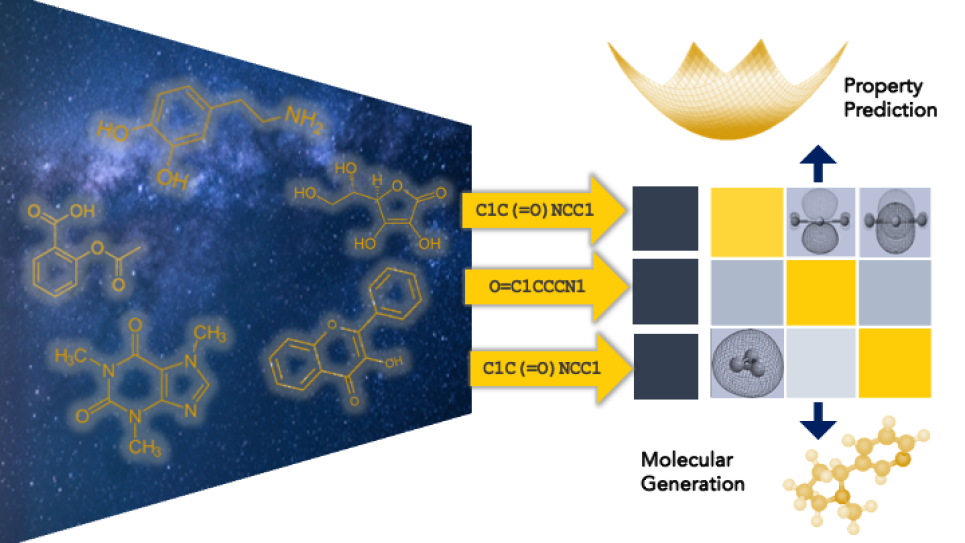
Foundation models for molecule design for energy storage applications. Image: Anoushka Bhutani, University of Michigan.
This project will scale up the training of foundation models to the largest available chemical libraries to advance the design of new electrolytes for energy storage and energy conversion applications.
Energy storage and conversion devices require electrolyte materials that enable functionality for various applications. Designing electrolytes for electric vehicles, grid storage, and electric aircraft presents a myriad of unique challenges for each target application. It is critical to design domain-specific energy storage materials to electrify transportation and industrial processes to create a sustainable and electrified future.
Current energy storage materials development is hindered by expensive experiments that limit exploration to a small set of known synthesizable and readily available materials. To address the unique demands of developing batteries for electric mobility and grid storage applications, such as high energy density, low cost, safety, scalability, and power density, it is essential to explore a much larger chemical space than the few hundred currently considered. Foundation models offer a solution to both the exploration and evaluation issues: these models use self-supervised pre-training strategies to leverage unlabeled datasets and learn representations of data that can be applied to downstream tasks. Large unlabeled datasets of billions of synthesizable molecules are readily available. This INCITE project will scale up the training of foundation models to the largest available chemical libraries to achieve accuracy similar to quantum mechanical computational methods and apply these to the electrolyte design problem.
The foundation models will be embedded into the team’s automated materials design workflow, AutoMat, to develop new energy storage materials for electric mobility and grid storage applications. Designing a good electrolyte involves optimizing for multiple properties (e.g., conductivity, voltage stability) over a large, high-dimensional design space. The team will investigate how emergent behavior observed in large-scale training of foundation models can be leveraged to posit better electrolyte compositions. The team’s work holds tremendous potential for revolutionizing electrolyte design and advancing the fields of energy storage and transportation.