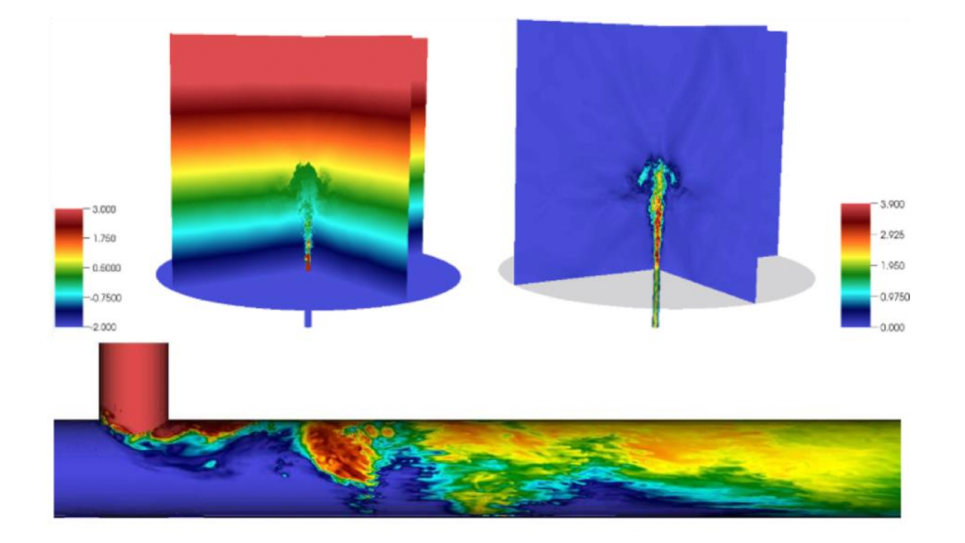
Setup for buoyancy-driven flow simulations to be studies: (top) DNS of buoyancy driven plume in stratified environment, showing the plume accelerating and then stabilizing into the neutrally buoyant layer [7]. (bottom) High-fidelity LES of thermal mixing at a pipe T-junction [8].