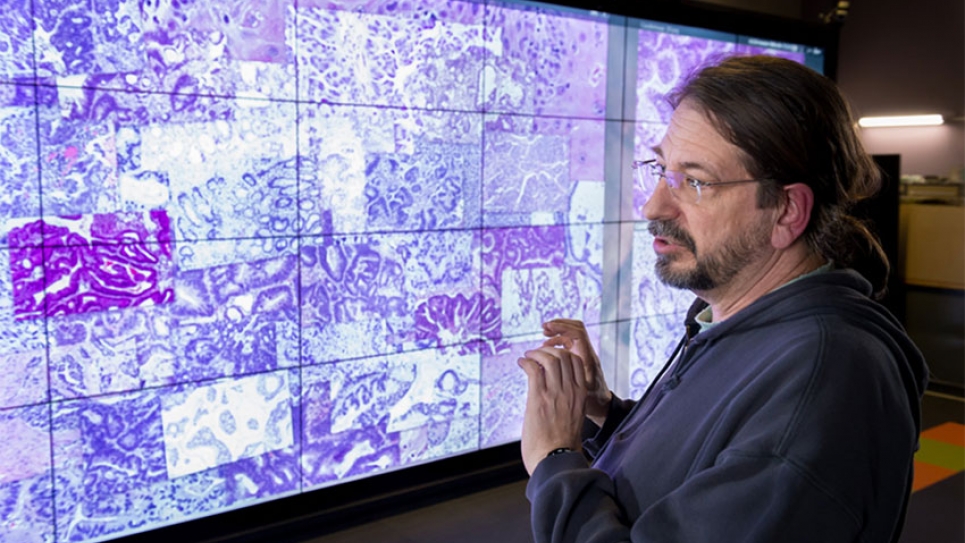
A cancer diagnosis is overwhelming, the treatment often complex and uncertain. Doctors have yet to understand how a specific cancer will affect an individual, and a drug that may hold promise for one patient, may not work for another.
But a melding of medical research and high-performance computing is taking a more personalized approach to treatment by creating precise therapy options based on genetics. "We are trying to devise a means of automating the search through machine learning so that you’d start with an initial model and then automatically find models that perform better than the initial one."
“Precision medicine is the ability to fine tune a treatment for each patient based on specific variations, whether it’s their genetics, their environment or their history. To do that in cancer, demands large amounts of data, not only from the patient, but the tumor, as well, because cancer changes the genetics of the tissue that it surrounds,” said Rick Stevens, Associate Laboratory Director for Computing, Environment and Life Sciences for the U.S. Department of Energy’s (DOE) Argonne National Laboratory.
In a typical cancer study today, more than eight million measurements are taken from the biopsy of a single tumor. But even as current technologies allow us to characterize the biological components of cancer with greater levels of accuracy, the massive amounts of data they produce have out-paced our ability to quickly and accurately analyze them.
To tackle these complicated and consequential precision medicine problems, researchers globally are looking toward the promise of exascale computing. Stevens is principal investigator of a multi-institutional effort advancing an exascale computing framework focused on the development of the deep neural network code CANDLE (CANcer Distributed Learning Environment).
Part of the Joint Design of Advanced Computing Solutions for Cancer (JDACS4C), a DOE and National Cancer Institute (NCI) collaboration, CANDLE will address three key cancer challenges to accelerate research at the molecular, cellular and population levels.
The challenges will test CANDLE’s advanced machine learning approach—deep learning—that, in combination with novel data acquisition and analysis techniques, model formulation and simulation, will help arrive at a prognosis and treatment plan designed specifically for an individual patient.
“Deep learning is the use of multi-layered neural networks to do machine learning, a program that gets smarter or more accurate as it gets more data to make predictions. It’s very successful at learning to solve problems,” said Stevens.
The model stores data that has already been observed and uses it later to quickly infer the solution to similar or recurring events or problems. Speech recognition, image recognition and text translation are examples of machine learning that many of us utilize every day without realizing it.
“Every time you talk to SIRI or Alexa, you’re encountering deep learning,” he added.
This framework will be built upon available open-source deep learning platforms that can be adapted to address different aspects of the cancer process as represented by JDACS4C’s challenge topics: 1) understand the molecular basis of key protein interactions; 2) develop predictive models for drug response; and 3) automate the extraction and analysis of information from millions of cancer patient records to determine optimal cancer treatment strategies.
The process begins by compiling all the known data on how cancer functions, reacts to drugs and behaves within individuals, and creating a virtual approximation of it. While the numbers of molecular configurations, drug combinations and patient datasets are staggering, the exascale-anticipating framework will progressively “learn” to manage it.
For example, the goal of the drug response challenge is to predict how a tumor will respond to a drug based on the characteristics of both the tumor and the drug, the information for which is identified through previously available data, such as tumor samples and previous drug screens.
The CANDLE network code will be trained to assimilate millions of previous drug screen results. An open-source content management system then would search through upwards of a billion drug combinations to find those with the greatest potential to inhibit a given tumor, or a billion hypothetical compounds to identify candidates for new drug development.
Through another technique called data mining, researchers working on the treatment strategy problem can train the network to sift through and automatically interpret millions of clinical reports and patient records. From those, it can pull data related directly to a specific patient and build predictive models of treatment and outcome trajectories for that individual.
Until now, cancer researchers have been doing this in small teams, maintaining massive databases of different factors characteristic of the cancer’s growth. But much of this information is peripheral. The most helpful information is buried within and among the millions of data points collected.
“This is a huge part of the challenge, because humans do this now, but by hand,” explained Stevens. “We are trying to devise a means of automating the search through machine learning so that you’d start with an initial model and then automatically find models that perform better than the initial one. We then could repeat this process for each individual patient.”
While the computational solutions for these training problems alone will require the largest available high-performance computers, Stevens and his team believe that the resulting models are likely to require exascale or near-exascale systems to advance each of the cancer problem areas.
CANDLE is one of three unique Argonne National Laboratory programs funded by the DOE’s Exascale Computing Project (ECP), launched in 2015 to promote the design and integration of application, software and hardware technologies into exascale systems.
These systems will be able to run applications such as CANDLE 50 to 100 times faster than today’s most powerful supercomputers, like those housed at the Argonne Leadership Computing Facility (ALCF) a DOE Office of Science User Facility. Theta, ALCF’s new 9.65 petaflops Intel-Cray system, delivers high performance on traditional modeling and simulation applications and was developed to more quickly and efficiently handle advanced software and data analysis methods.
“The types of things researchers would like to accomplish now require a lot more data, capacity and computing power than we have. That’s why there is this effort to build a whole new framework, one focused more on data,” said Paul Messina, director of ECP. “CANDLE will play an essential role in the development of applications that drive this framework, creating the ability to analyze hundreds of millions of items of data to come up with individual cancer treatments.”
With the unique collaboration of JDACS4C, the CANDLE team has immediate access to NCI’s formidable subject matter and domain experts on cancer. And as partners with the DOE and, specifically, CORAL (a collaboration comprising Oak Ridge, Argonne and Lawrence Livermore National Laboratories), CANDLE enlists some of the nation’s leading computational scientists to provide the computational and data science expertise.
Vendors involved with the labs and ECP are among the leading designers of high-performance computing architecture in the world. Companies like Intel, Nvidia, IBM, and Cray are interested in collaborating on cancer research, and are fully vested in the idea that the convergence between simulation, data and machine learning is the future, noted Stevens.
“There is a tremendous level of team work and sharing across the enterprise. Cancer is something that people can relate to personally, so having the opportunity to develop a capability that will eventually help somebody else can be very motivating,” said Eric Stahlberg, director of the Frederick National Laboratory for Cancer Research’s strategic and data science initiatives.
“It’s a Herculean task. But even incremental progress toward that goal will have a significant impact on many more people affected by cancer, as a result.”
Argonne National Laboratory seeks solutions to pressing national problems in science and technology. The nation's first national laboratory, Argonne conducts leading-edge basic and applied scientific research in virtually every scientific discipline. Argonne researchers work closely with researchers from hundreds of companies, universities, and federal, state and municipal agencies to help them solve their specific problems, advance America's scientific leadership and prepare the nation for a better future. With employees from more than 60 nations, Argonne is managed by UChicago Argonne, LLC for the U.S. Department of Energy's Office of Science.
The U.S. Department of Energy's Office of Science is the single largest supporter of basic research in the physical sciences in the United States and is working to address some of the most pressing challenges of our time. For more information, visit the Office of Science website.