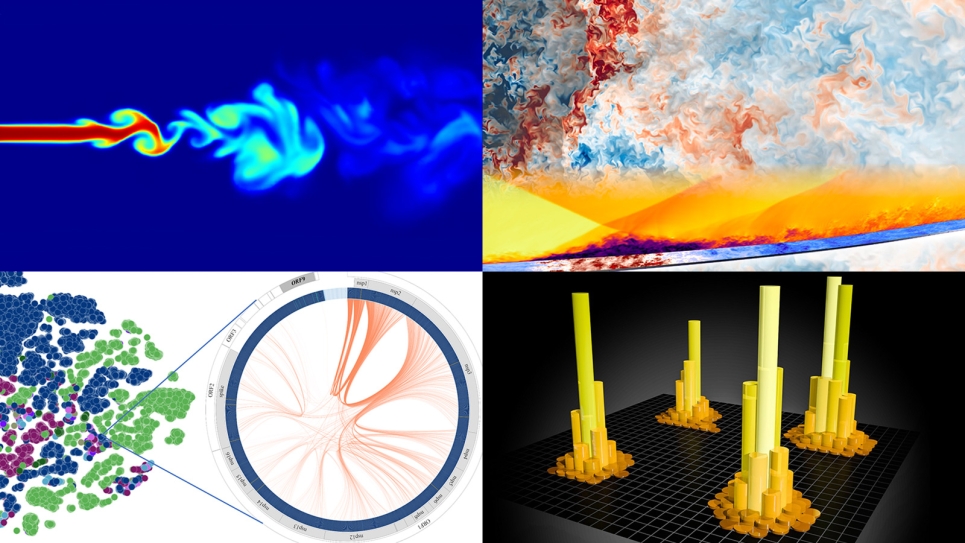
Over the past year, the ALCF user community has produced a number of groundbreaking studies in areas ranging from climate modeling to COVID-19 research to materials design.
In 2022, scientists from across the world used supercomputing and artificial intelligence (AI) resources at the U.S. Department of Energy’s (DOE) Argonne National Laboratory to accelerate discovery and innovation across a wide range of research areas.
From using AI to study the evolutionary dynamics of COVID-19 to developing a new method to assess the likelihood of extreme droughts, research projects supported by the Argonne Leadership Computing Facility (ALCF), a DOE Office of Science user facility, continued to push the boundaries of scientific computing.
The ALCF also had a notable year in rolling out new high performance computing (HPC) resources to the research community, with the deployment of Polaris and the first ALCF AI Testbed systems. The facility also made considerable strides in standing up its next-generation exascale supercomputer, Aurora.
As 2022 draws to a close, we take a look back at some of the groundbreaking research campaigns carried out by ALCF users over the past year. For more examples of the scientific advances enabled by ALCF computing resources, check out the 2022 ALCF Science Report.
An Argonne-led team was recognized with the 2022 Gordon Bell Special Prize for High Performance Computing-Based COVID-19 Research for their efforts to create the first genome-scale language models (GenSLMs) to study the evolutionary dynamics of SARS-CoV-2. The researchers used the ALCF’s Polaris system and Cerebras CS-2 AI platform to develop large language models that can track genetic mutations in SARS-CoV-2 and predict variants of concern. The team's research has the potential to transform how scientists identify and classify new and emergent variants of SARS-CoV-2 and other pandemic-causing viruses.
A team led by scientists from the University of Michigan used ALCF computing resources to advance tomographic reconstruction algorithms for materials characterization research. In one of their studies, the team developed a limit-pushing method for creating high-resolution visualizations of atomic structure. Known as fused multi-modal electron microscopy, the technique allows for high signal-to-noise ratio recovery of nanomaterial chemistry, providing a tool that can help scientists peer inside chemical systems to explore their structure and behavior at the microscopic level.
Combining experimental work with simulations, an Argonne team developed a novel battery electrolyte that can hold significantly more water than conventional electrolytes. Building on results obtained in laboratory experiments, scientists performed simulations on the ALCF’s Theta supercomputer to get an atomic-scale view of the underlying chemical mechanisms that give rise to favorable battery performance. Their simulations revealed that the material, composed of a lithium salt and an ionic liquid, could separate and bind up water, sequestering individual water molecules. The team’s study provides a potential pathway for battery manufacturers to incorporate water in the battery fabrication process, allowing for lower-cost, more environmentally friendly batteries.
With support from DOE's HPC4EI program, researchers from 3M partnered with Argonne to leverage the lab's AI and supercomputing resources to improve the energy efficiency of a manufacturing process used to produce melt-blown nonwoven materials, which are essential to N95 masks and a range of other filters and fabrics. The team carried out a series of simulations on the ALCF’s Theta supercomputer to develop an initial model of the melt-blowing process. Their optimization approach combined machine learning for simulation parameter selection with high-fidelity fluid dynamics simulations for modeling the process and nozzle geometry. Using data generated from the simulations, the machine learning algorithm suggests successive simulations with parameter settings iteratively refined to minimize energy consumption. The team will use their findings to zero in on an optimal design that can reduce energy consumption by 20% without compromising material quality.
With help from the ALCF’s Theta supercomputer, Argonne researchers developed a new method to assess the likelihood of extreme drought conditions across the United States. Instead of using precipitation deficit, the team turned to a new measure called vapor pressure deficit to help identify short-term droughts and predict future extreme droughts, including “flash drought” events with a quick onset period that could be as short as few weeks. Using this method, based on highly detailed regional climate models, they have found that droughts are likely to be exacerbated by global warming.
Phase diagrams are an invaluable tool for materials synthesis, providing researchers with information on the phases of a material at any given thermodynamic condition (e.g., pressure, temperature, chemical composition). To extend their utility to a promising but mysterious class of metastable materials, Argonne researchers leveraged ALCF computing resources and machine learning to develop an automated workflow to construct metastable phase diagrams. Using carbon as a prototypical system, the team demonstrated how their machine learning approach could be used to create phase diagrams that map hundreds of metastable states ranging from near equilibrium to far-from-equilibrium. Applying the machine learning algorithm to more complex systems could lead to broad impact on the discovery and design of useful metastable materials.
A team led by researchers from the University of Maryland used ALCF supercomputing resources to advance our understanding of supersonic turbulent boundary layers and their interaction with shock waves. The team used the ALCF’s Theta system to perform direct numerical simulations of shock/boundary-layer interactions with crossflow to approximate the off-design operating conditions that can ultimately cause aircraft engine performance degradation and flameout. By providing new insights into the complex physics of airflow, the team’s research aims to improve modeling techniques that can help improve the performance of supersonic and hypersonic aircraft.
Using the ALCF’s supercomputing and AI resources, an Argonne team developed a new method to speed up the analysis of data generated from high-energy X-ray experiments. The researchers demonstrated that their neural network-based method called BraggNN can more precisely identify Bragg peaks — data points that indicate positions and orientations of tiny individual crystals — in a fraction of the time they used to. The team used ALCF’s Theta and Cerebras CS-2 systems to rapidly train their deep learning model, which was able to deliver sub-pixel accuracy in determining the peak positions.
As part of an Aurora Early Science Program project, researchers used the ALCF’s Groq AI system to explore how deep learning-guided investigations could help inform the operation of future fusion energy devices. Due to the real-time inference demands of tokamak fusion reactors, the team turned to the AI platform to enable fixed, predictable compute times for inference that would vary in duration if carried out on conventional HPC systems. Ultimately, the researchers aim to develop a workflow that uses supercomputers for AI training and AI accelerators for inference.
Over the past year, Argonne researchers have advanced the use of principles that could make scientific data more findable, accessible, interoperable, and reusable, or FAIR, for both people and machines. In one study, the team laid out a set of new practices to guide the curation of high energy physics datasets to help researchers make them more FAIR. In another effort, the researchers used the ALCF's Theta and SambaNova DataScale systems to demonstrate how to create and share FAIR data and AI models within a unified computational framework. By sharing best practices for using FAIR AI priniciples, the team's work aims to accelerate new discoveries by making it easier for researchers to explore and reuse existing scientific datasets.
Researchers from University College London combined physics-based simulations with machine learning methods at the ALCF to speed up the search for promising new drugs to combat COVID-19 and other viruses. The team’s simulations determine the physical properties of compounds to use as training data for the machine learning algorithm, which then predicts potentially better molecules, which in turn are analyzed using physics-based simulations and then given back to the machine learning algorithm. This continues in a loop until it produces a short list of compounds likely to bind with the protein. The team’s work has resulted in multiple publications, including a study that employs their Thermodynamic Integration with Enhanced Sampling (TIES) method to automate the process of setting up, running, and analyzing relative binding free energy calculations. As part of their project, the team also collaborated with researchers across the world to develop a drug discovery pipeline called IMPECCABLE (Integrated Modeling PipelinE for Covid Cure by Assessing Better Leads) that is being used to search for drugs to treat COVID-19.
==========
The Argonne Leadership Computing Facility provides supercomputing capabilities to the scientific and engineering community to advance fundamental discovery and understanding in a broad range of disciplines. Supported by the U.S. Department of Energy’s (DOE’s) Office of Science, Advanced Scientific Computing Research (ASCR) program, the ALCF is one of two DOE Leadership Computing Facilities in the nation dedicated to open science.
Argonne National Laboratory seeks solutions to pressing national problems in science and technology. The nation’s first national laboratory, Argonne conducts leading-edge basic and applied scientific research in virtually every scientific discipline. Argonne researchers work closely with researchers from hundreds of companies, universities, and federal, state and municipal agencies to help them solve their specific problems, advance America’s scientific leadership and prepare the nation for a better future. With employees from more than 60 nations, Argonne is managed by UChicago Argonne, LLC for the U.S. Department of Energy’s Office of Science.
The U.S. Department of Energy’s Office of Science is the single largest supporter of basic research in the physical sciences in the United States and is working to address some of the most pressing challenges of our time. For more information, visit https://energy.gov/science.