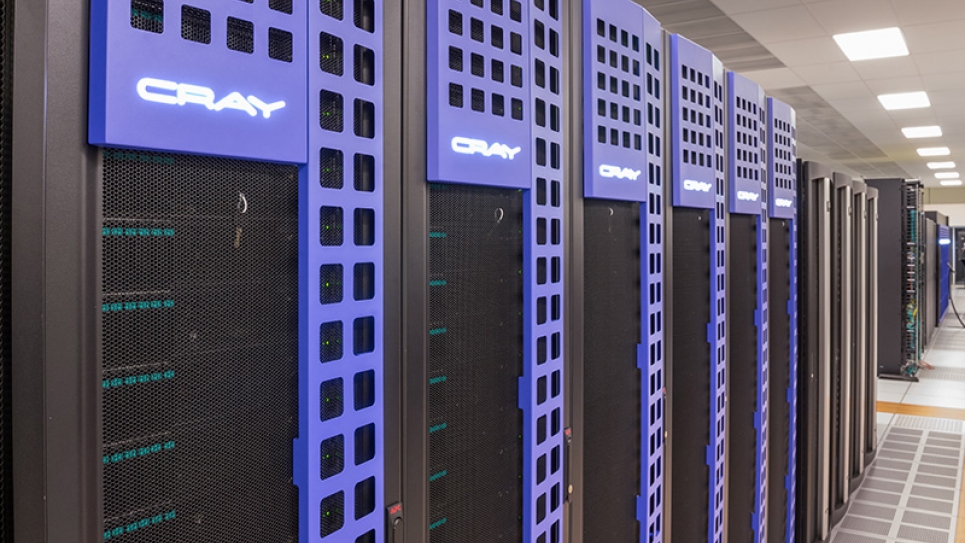
ALCF-SWIFT team recognized for their work to use HPC and AI to enable a more secure worldwide financial infrastructure.
As part of the 2023 Commercialization Excellence Awards at the U.S Department of Energy’s (DOE) Argonne National Laboratory, researchers from the Argonne Leadership Computing Facility (ALCF) were recognized with a Delivering Impact Award for their collaboration with the Society for Worldwide Interbank Financial Telecommunication (SWIFT) to enable a more secure worldwide financial infrastructure. The ALCF is a DOE Office of Science user facility at Argonne.
SWIFT is a global provider of financial messaging services, facilitating over 95 percent of interbank financial transactions across the planet and underpinning the American and worldwide financial systems. In this capacity, SWIFT processes more than 8.4 billion messages a year and interconnects over 11,000 institutions in more than 200 countries.
Supported by a Cooperative Research and Development Agreement (CRADA) between SWIFT and Argonne, the collaborative project explores the use of the ALCF’s artificial intelligence (AI) and high-performance computing (HPC) resources for the examination of SWIFT’s data streams. The ALCF team includes Venkatram Vishwanath, Michael E. Papka, William Allcock and Filippo Simini.
The team’s research takes a data-driven approach and employs novel AI methods to explore SWIFT’s synthetic transactional data streams and identify patterns, including any anomalous activity. Generative AI approaches developed by the team enable the creation of synthetic datasets with spatial and temporal characteristics similar to SWIFT transaction streams.
Given that SWIFT’s large data volumes and high data rates necessitate the use of HPC systems, the team is leveraging the ALCF’s powerful supercomputing resources to aid the analysis of the data stream. These resources enable significantly larger-capacity datasets to be processed at higher rates, as well the simulation of real-world SWIFT scenarios. The pattern-detection methods developed by the ALCF team will enable SWIFT to respond to anomalies more rapidly, thereby strengthening financial infrastructure.
The development of robust AI methods for analysis of high volumes of streaming data is of importance to DOE in research domains spanning imaging, high-energy physics, manufacturing, and more.