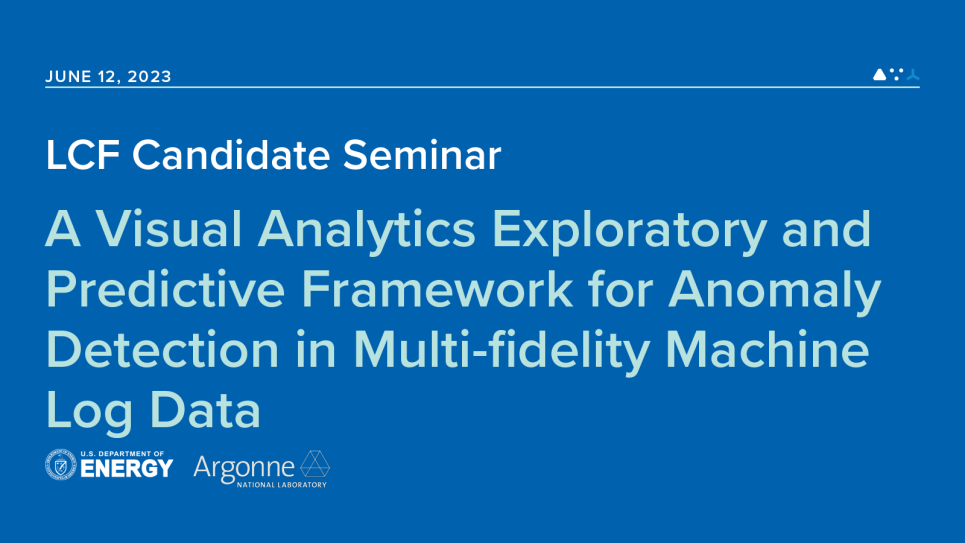
Maintaining robust and reliable computing systems, especially those enabling breakthrough work in computational science and engineering research, is critical and challenging. Therefore, we build an exploratory mechanism for pattern identification from historical data and a predictive tool for identifying the large-scale systems' state with a visual analytics framework. The work introduces (1) incremental and progressive functional data analysis (FDA) to process time-dependent phenomena promptly; (2) a scalable visual analytics tool to effectively characterize system behavior and faults over time; (3) visual analytics machine learning pipeline to promptly predict a user application's exit status and potential errors; and (4) visual analytics solution for data exploration at varying temporal and spatial resolutions using multiresolution dynamic mode decomposition (mrDMD). Thus building faster, scalable, and interactive visual analytics solutions utilizing exploratory, predictive, and multiresolution analyses of diverse large-scale system logs to bridge the gap between visual analytics and machine log analysis.
To add to calendar:
Click on: https://wordpress.cels.anl.gov/cels-seminars/
Enter your credentials.
Search for your seminar
Click “Add to calendar”