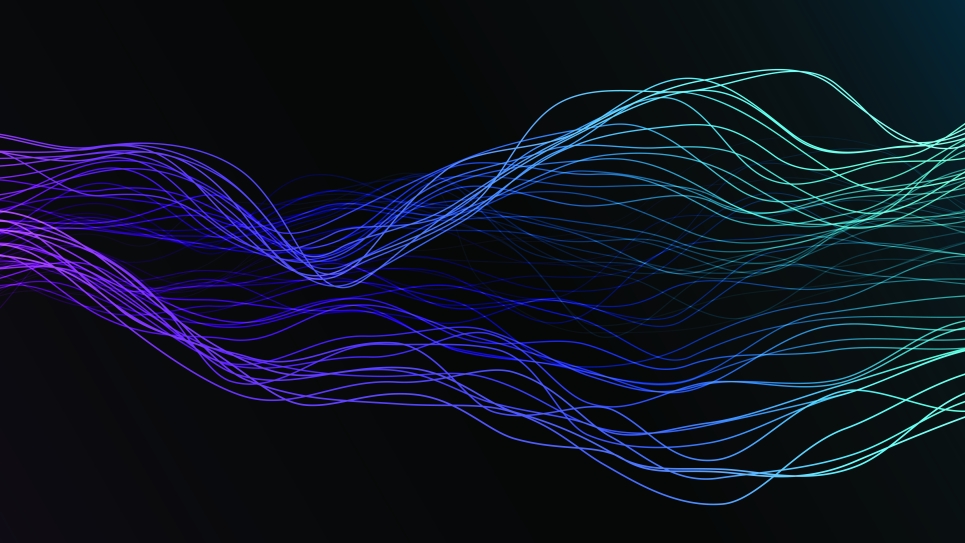
Developing reduced-complexity models which can represent complex systems in an optimal low-dimensional space, be used to develop predictive models with reduced computational expense, and further uncover underlying dynamical information has become increasingly popular within the field of fluid mechanics. These models may be developed from either a physics-based approach, in which the governing equations are utilized, or from data-driven methods, in which an array of training data for the system might be available. This talk discusses the use of data-driven and physics-based modeling methodologies to capture and predict complex fluid dynamical behavior for problems relevant to the field of aerospace and mechanical engineering, such as active flow control for unsteady aerodynamic configurations and the multi-phase nature of flows pertaining to fuel injection, to demonstrate the wide variety of applications that reduced-complexity modeling methods can address.
Data-driven and physics-based modeling methodologies are employed to capture and predict the response to burst-type momentum-injection actuation near the leading edge of a stalled NACA0009 airfoil, utilizing data from direct numerical simulations. The response to this type of actuation can be decomposed into two components: a short-time response that is characterized by an initial decrease followed by an increase in the lift, and a long-time response that can be sensitive to the instantaneous wake state at the onset of actuation. Theoretical models are developed using the assumptions from classical unsteady aerodynamic theory, which provide insight into the form that the data-driven models take. The data-driven models, which are identified using variants of dynamic mode decomposition (DMD), can capture both the short- and long-time response of the system to actuation. Capturing the unsteady interactions inherent to the wakes of bluff bodies can be quite complex depending on the geometrical configuration and associated Reynolds number. A physics-based approach is utilized to extract spectral content from systems with limited data available, and the methodology developed is applied to wake flows with dominant vortex interactions for flows with increasing complexity. Finally, we discuss the application of modal decomposition methods to flows of the multi-phase nature, where techniques such as proper orthogonal decomposition (POD) can provide insight into how changes to a fuel injector geometry influence the leading dominant spatial mode features of the flowfield internal to the injector, as well as variations in the modal energy content and distribution. Concluding results further illustrate the application of these methods to flows of a multi-phase nature, where DMD can supplement information relevant to the break-up of a liquid jet in a cross-flow environment, typical of after-treatment systems, to develop informed computational fluid dynamical simulations of the spray breakup.
Zoom Link: https://argonne.zoomgov.com/j/1606228075
See all upcoming talks at http://wordpress.cels.anl.gov/lans-seminars/