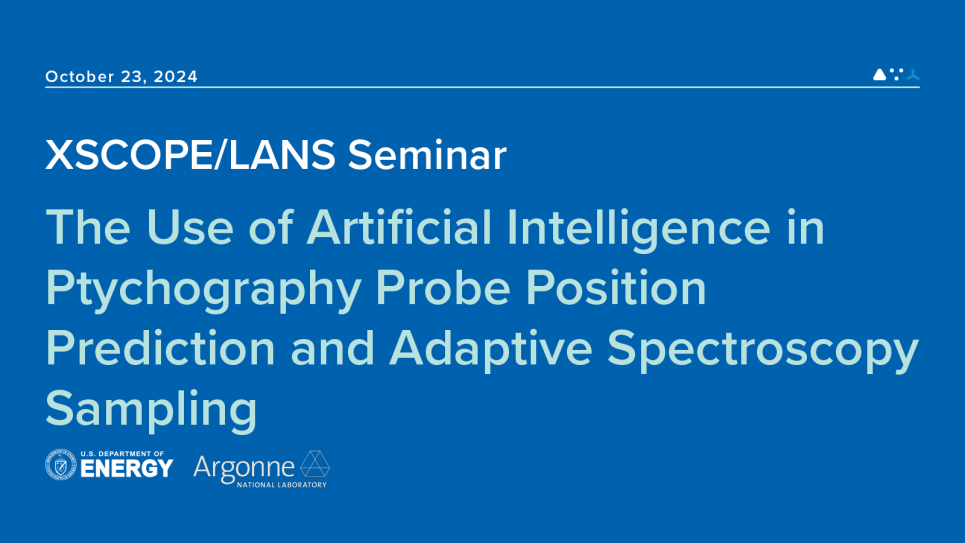
Artificial intelligence has transformed the research and operations done at synchrotron light sources in many ways. To name a few, it offers the possibility to solve inverse problems without traditionally essential constraints and conditions as an AI model learns the prior of solution distribution through data. Also, when used in the domain of autonomous experimentation, it can guide the acquisition of data in a more efficient manner without human intervention. In this talk, I will present AI-based solutions illustrating each of the aforementioned areas of significance. First, I will introduce how we use the single-shot phase retrieval capability of PtychoNN, a ptychography reconstruction neural network, to predict the probe positions of ptychography using only diffraction patterns without any initial guess. Second, I will describe our work on developing a Bayesian optimization algorithm that guides the experimental data acquisition of XANES spectroscopy, with which a spectrum can be collected with an RMS error below 0.5% * (absorption edge height) compared to the densely sampled ground truth while measuring only 15 - 20% of the points.
Bio: Ming Du is an assistant computational scientist in the Computation and Artificial Intelligence group of the Advanced Photon. Ming obtained his PhD in Materials Science and Engineering at Northwestern University in 2019. He was postdoc at Argonne National Laboratory between 2019 and 2021, working with Prof. Chris Jacobsen for both his PhD and postdoctoral research. He worked as a senior algorithm engineer at KLA Corporation from 2021 to 2023. His primary research interests include inverse problem solving in computational imaging such as ptychography and computed tomography, parallel computing of image reconstruction algorithms, and artificial intelligence for imaging and computer vision problems.
See all upcoming talks at https://www.anl.gov/mcs/lans-seminars