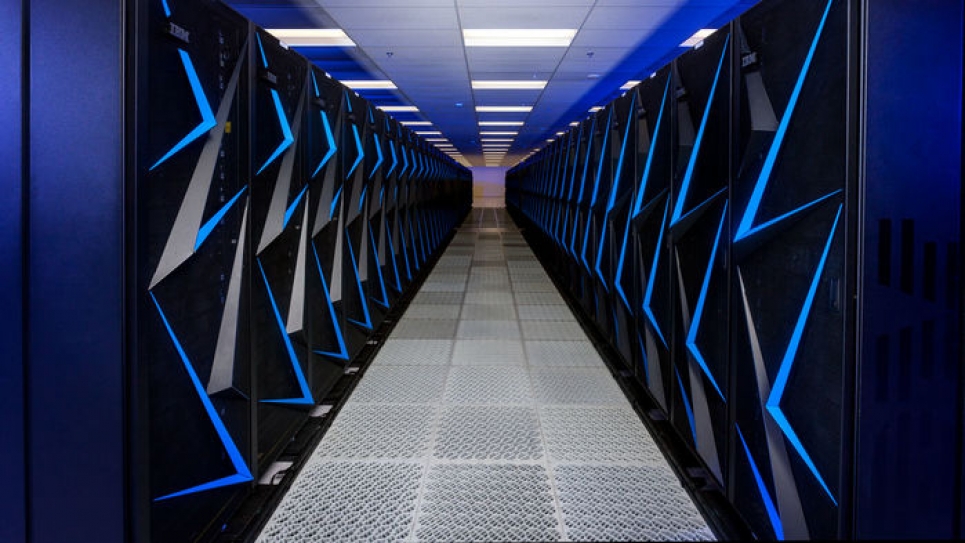
The amount of data being generated and gathered in scientific simulations is continuously growing, putting mounting pressure on storage and bandwidth limitations. New creative ways of handling the data movement bottleneck problem have been proposed, however, many of the solutions can lack the theoretical backing that ensures the validity of the solution. In this talk, we will review our ongoing efforts to provide theoretical support for adopting ZFP lossy compression as a new data-type for scientific simulations. ZFP is a lossy compression algorithm designed by Peter Lindstrom that was designed specifically for floating-point data. It decomposes the data set into blocks which are then compressed and decompressed independently. ZFP itself has been shown to be quite effective for I/O operations and in-memory storage of static data. However, there is potential for significant performance gains by using compressed data types within a simulation. As ZFP allows for small deviations from the original data is extremely important to understand how the error as well as the bias from lossy compression impacts the accuracy of the solution.
Zoom Link: https://argonne.zoomgov.com/j/1601604148