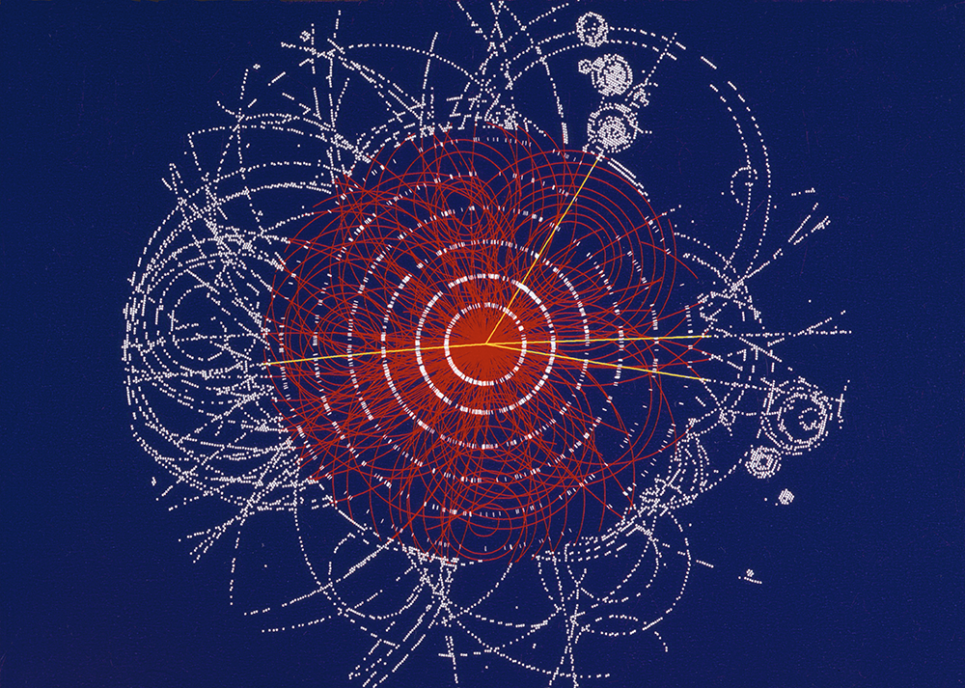
Topological data analysis (TDA) has been used to understand and summarize complex data in science and engineering, ranging from climate, neuroscience, to cosmology. However, scientific data's ever-increasing complexity poses grand challenges to traditional methodologies and necessitates for TDA to use statistical information and integrate tools from machine learning.
This talk covers two examples of using statistics in merge trees---a topological summarization of the structural information---for understanding, visualizing, and reducing scientific data. First, I will present a merge-tree-based comparative measure using labeled interleaving distances for scalar fields. Such merge tree comparison helps detect transitions, clusters, and periodicities for time-varying datasets. I will also demonstrate the structural average of a set of labeled merge trees for uncertainty visualization. Second, I will illustrate the use of merge trees to quantify the structural stability of vector field features to perturbations. Specifically, the multi-level robustness for the topological analysis of 2D vector fields data can enhance feature tracking, selection, and comparison in E3SM simulations and ERA5 reanalysis data.
Bio: Lin Yan is a PhD student in the Scientific Computing and Imaging (SCI) Institute, University of Utah. She received BS and MS degrees in control science and engineering from Shanghai Jiao Tong University in 2010 and 2013, respectively. Her research interests include topological data analysis and visualization. Her recent work focuses on problems involving large and complex forms of data by combining topological, statistical data analysis and visualization techniques.
Join ZoomGov Meeting
https://exascaleproject.zoomgov.com/j/1614084302
Meeting ID: 161 408 4302
One tap mobile
+16692545252,,1614084302# US (San Jose)
+16468287666,,1614084302# US (New York)