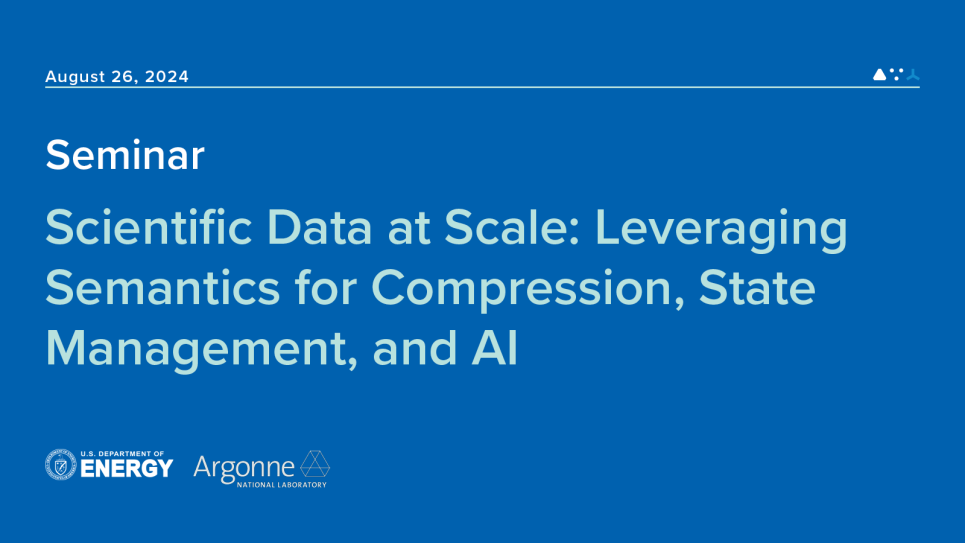
Data is foundational to our understanding of our world, and this is especially true in science we use data to study and assess hypotheses that test our understanding of the world. To really leverage AI for Science, we need an approach that allows us to incorporate the breadth of scientific data we use across the lab while not requiring a "heroic" effort for each application. In this talk, Robert will present his contributions understanding scientific data over the course of his postdoc at Argonne, presents a technical deep dive into his recent work Evostore which leverages checkpoints to accelerate neural architecture search which could be used to accelerate finding new architectures to leverage multi-modal science data, and presents his vision on how to leverage multi-modal data in AI.
Bio:
Robert Underwood is a Post Doctoral Appointee in the Mathematics and Computer Science Division at Argonne National Laboratory focusing on using data compression to accelerate I/O for large-scale scientific applications including AI for Science. He currently co-leads the AuroraGPT Data Team with Ian Foster. In addition to AI, Robert's library LibPressio, allows users to experiment and adopt advanced compressors quickly, has over 200 average unique monthly downloads, is used in over 17 institutions worldwide, and he is also a contributor to the R&D100 winning SZ family of compressors and other compression libraries. He regularly mentors students and is the early career ambassador for Argonne to the Joint Laboratory for Extreme Scale Computing.