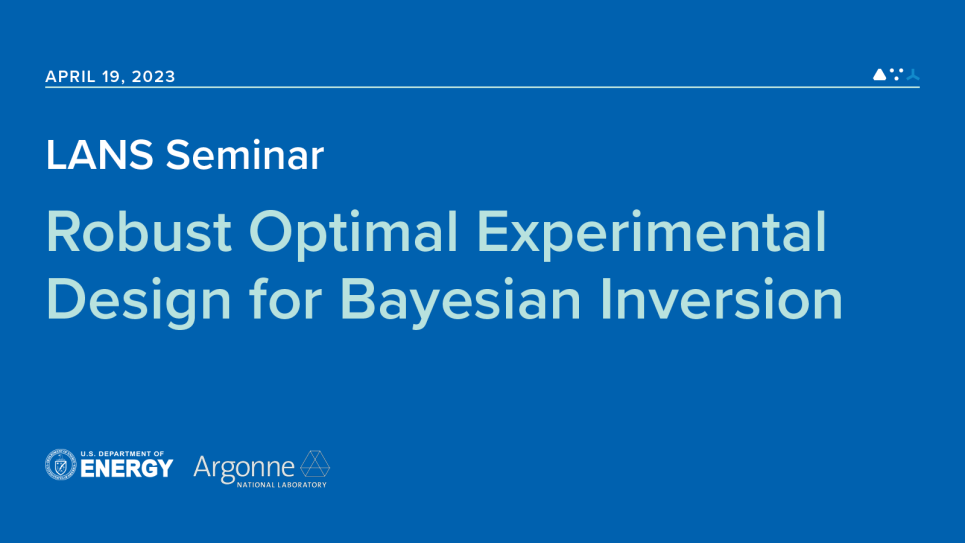
Optimal data acquisition, for inverse problems, can be modeled as an optimal experimental design (OED) problem, which has gained wide popularity and attention from researchers in various fields in statistics, engineering, and applied math. Challenges in model-constrained OED include high-dimensionality of the underlying inverse problem, misrepresentation of uncertainties and experimental setup, among others. In this talk, we provide an overview of our recent developments in the area of model-constrained OED and optimal sensor placement. Specifically, we discuss an efficient stochastic learning approach for solving challenging binary optimization problems ideally suited for, but are not limited to, solving challenging binary OED problems for sensor placement. Additionally, we discuss efficient approaches for robustifying the solution of such binary OED optimization problems.
Bio: Ahmed Attia received his B.S. degree in Mathematics, Statistics and Computer Science in 2004, and his M.S. degree in Statistics and Computer Science in 2008, from Mansoura University, Egypt. In 2016, he obtained his Ph.D. degree, in Computer Science and Applications from Virginia Polytechnic Institute and State University (Virginia Tech), USA. He worked as an intern, in 2014 and in 2015, at Argonne National Laboratory in the Mathematics and Computer Science department. In 2016, he worked as a postdoctoral research fellow at SAMSI, the Statistical and Applied Mathematical Science Institute (RTP, NC), and he was also affiliated with the Mathematics Department at North Carolina State University. In 2017, he joined Argonne National Laboratory (Lemont, IL) as a postdoctoral researcher in the Mathematics and Computer Science department and is currently an Assistant Computational Mathematician. Ahmed’s research interests are in the area of computational science and engineering.
See all upcoming talks at https://www.anl.gov/mcs/lans-seminars