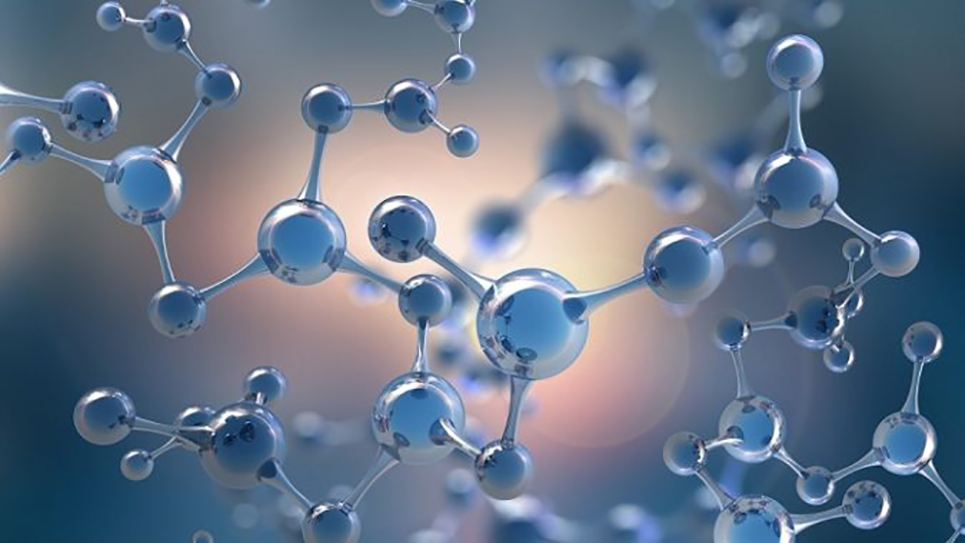
Automating molecular design with deep reinforcement learning (RL) can significantly accelerate the search for novel materials. Despite recent progress on leveraging graph representations to design molecules, such methods are fundamentally limited by the lack of three-dimensional information. In this talk, we present a novel RL formulation for molecular design in Cartesian coordinates to extend the class of explorable molecules. Our reward function is based on fundamental physical properties, which we approximate via quantum-chemical methods. We propose a novel actor-critic architecture that exploits the symmetries of the design process through a rotationally covariant state-action representation based on a spherical harmonics series expansion. To enable progress towards de-novo molecular design, we introduce MolGym, an RL environment comprising several challenging molecular design tasks along with baselines. Our experiments show that our agent can efficiently learn to solve molecular-design tasks from scratch that are unattainable with graph-based approaches.
Bio: Gregor Simm is a research associate working with José Miguel Hernández-Lobato in the Machine Learning Group at the University of Cambridge. His interests are in Reinforcement Learning, Graph Neural Networks, and the application of machine learning to physical sciences. Gregor's research is supported by an Early Postdoc.Mobility fellowship of the Swiss National Science Foundation. See Gregor's CV and publications for more information.