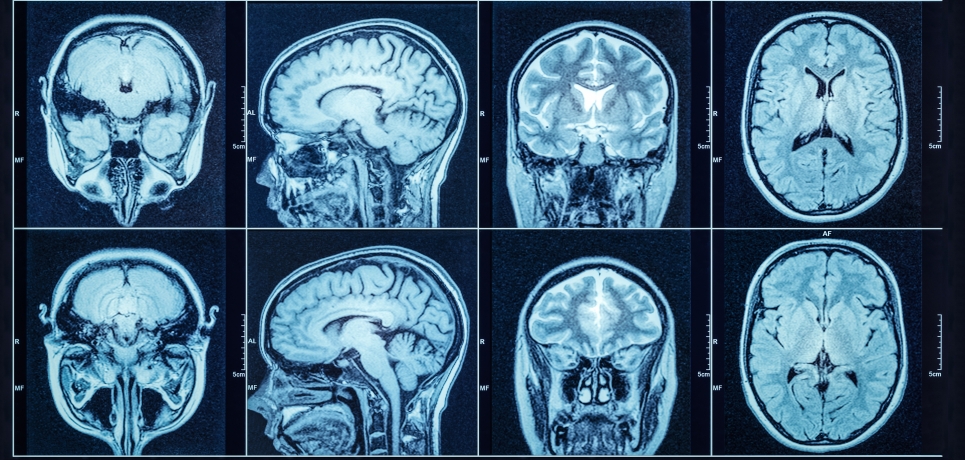
Abstract: It is often said that an image is worth a thousand words. However, the size of raster images is in megabytes while a thousand words could be just a few kilobytes! As opposed to quantitative techniques, imaging / tomography has largely been used as a qualitative technique for visualization due to the computational challenges in reliable information extraction from large 3D image datasets with minimal supervision. This challenge is exacerbated at synchrotron facilities where imaging modalities are used in a vast application space. The advances in convolutional neural networks (CNN) for image analysis have facilitated parameter-free image-processing workflows for rapid data-reduction from multiple CT scans. In my talk, I will show two distinct use cases for quantitative imaging that I have developed for DOE-VTO and industry funded projects in fuel injection research: (1) time-resolved 3D imaging of flows inside opaque nozzles and (2) DL-based segmentation for metrology in micro-tomography. In the first case, I will discuss automation aspects of data acquisition and the benefits of near-real-time analysis for improving data quality. In the second case, I will discuss the development of an end-to-end 3D segmentation toolkit that deploys “sample-agnostic” 2D CNN models for surface determination (metrology) and particle analysis. Finally, I will outline a roadmap for AI-driven steering of imaging experiments.
Please use this link to attend the virtual seminar: