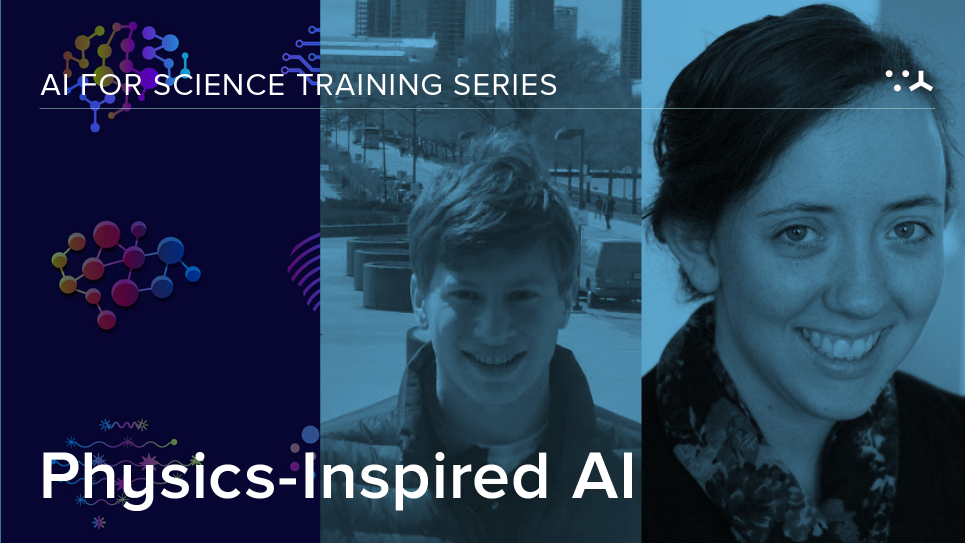
Trainees will be acquainted with physics-informed machine learning principles and applications, including physics-informed neural networks (PINNs) to solve forward and inverse problems; as well as their use to solve partial differential equations with a variety of boundary conditions.
Time: February 3, 3-5 p.m. US CT
This session is a part of the ALCF AI for Science Training Series.
Shawn Rosofsky is a graduate student at the University of Illinois at Urbana-Champaign pursuing a PhD in physics. His research involves simulating gravitational wave sources to help detectors such as LIGO identify and interpret the gravitational wave signals they observe. Shawn's current work is with the National Center for Supercomputing Applications' (NCSA) Gravity Group.
Dr. Bethany Lusch is an Assistant Computer Scientist in the data science group at the Argonne Leadership Computing Facility at Argonne National Lab. Her research expertise includes developing methods and tools to integrate AI with science, especially for dynamical systems and PDE-based simulations. Her recent work includes developing machine-learning emulators to replace expensive parts of simulations, such as computational fluid dynamics simulations of engines and climate simulations. She is also working on methods that incorporate domain knowledge in machine learning, representation learning, and using machine learning to analyze supercomputer logs. She holds a PhD and MS in applied mathematics from the University of Washington and a BS in mathematics from the University of Notre Dame.