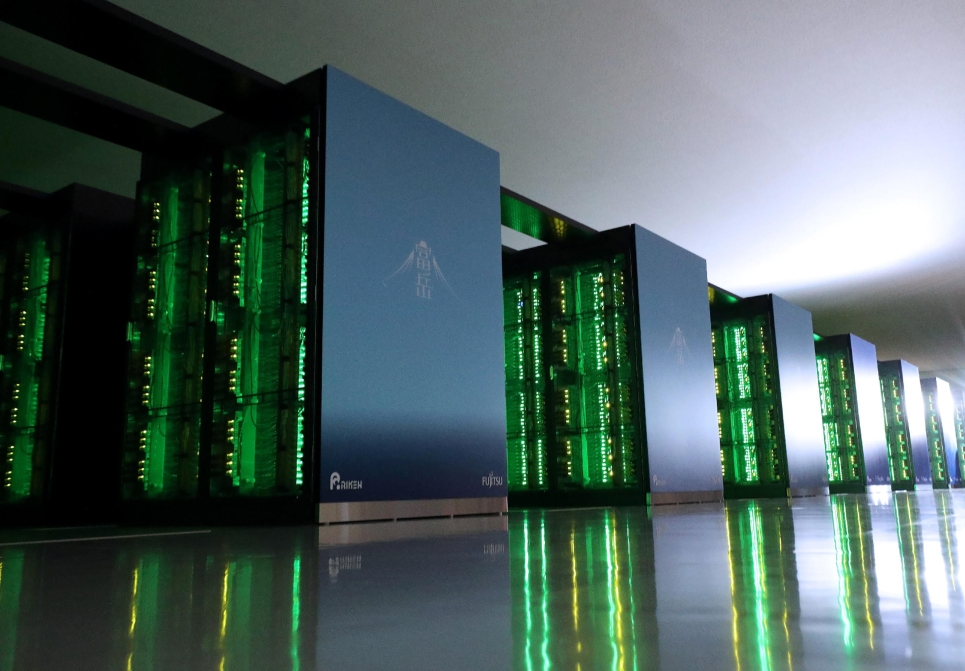
Traditional approaches for scientific computation have undergone remarkable progress but they still operate under stringent requirements such as they need precise knowledge of underline physical laws, precise knowledge of boundary and/or initial conditions, and often need time-consuming workflows such as mesh generation and long-time simulation. On top of these limitations, high-dimensional problems governed by parameterized PDEs cannot be tackled. Moreover, seamlessly incorporating noisy data is still a challenge for solving inverse problems efficiently.
Physics-Informed Machine learning (PIML) has emerged as a promising alternative for solving above mentioned problems. In this talk, we will discuss a particular type of PIML method namely, Physics-Informed Neural Networks (PINNs). We review some of the current capabilities and limitations of PINNs and discuss diverse applications where PINN is proved to be very effective compared to traditional approaches. We also discuss the extensions of the current PINN method such as Conservative PINNs (cPINNs) and eXtended PINNs (XPINNs) for big data and/or large models. To this end, we will also discuss various adaptive activation functions that can accelerate the convergence of deep and physics-informed neural networks.
Zoom Link: https://argonne.zoomgov.com/j/1618625918