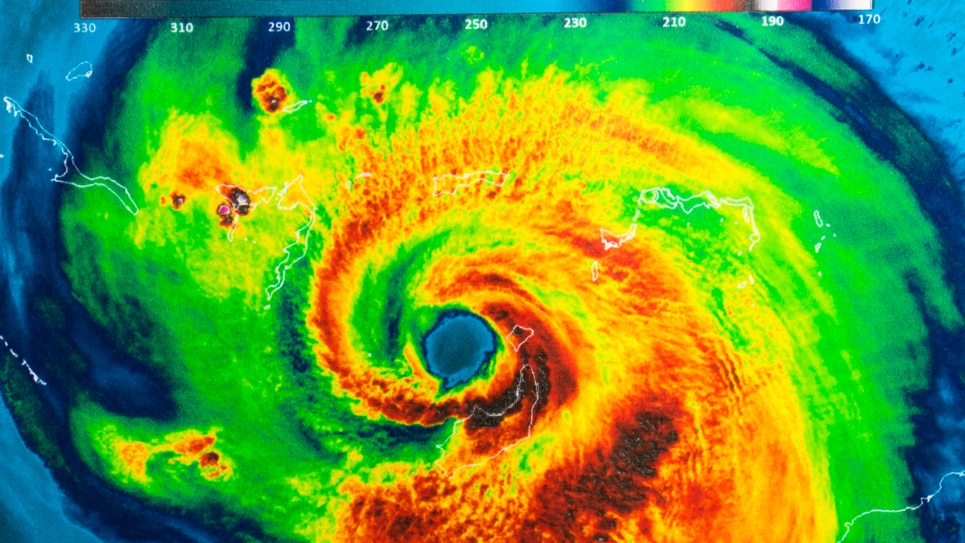
Machine learning (ML) has been shown to successfully predict the 3-dimensional atmosphere, however, a purely data-driven model does not include physical constraints such as the conservation laws. Numerical weather prediction models, on the other hand, use the physics-based governing equations to evolve the atmosphere and have become the backbone of operational weather prediction for the last several decades. However, they rely on parameterizations for sub-grid scale processes which can introduce biases.
We assess the performance of a hybrid modeling approach based on combining an atmospheric general circulation model (AGCM) with a parallel machine learning algorithm. The optimal flow-dependent combination of the two components is determined by training on ERA5 reanalyses. The hybrid model produces more accurate weather forecasts for the first 8 days. We also demonstrate that the hybrid model can simulate the Earth’s climate with substantially smaller systematic errors and more realistic variability than the host AGCM.