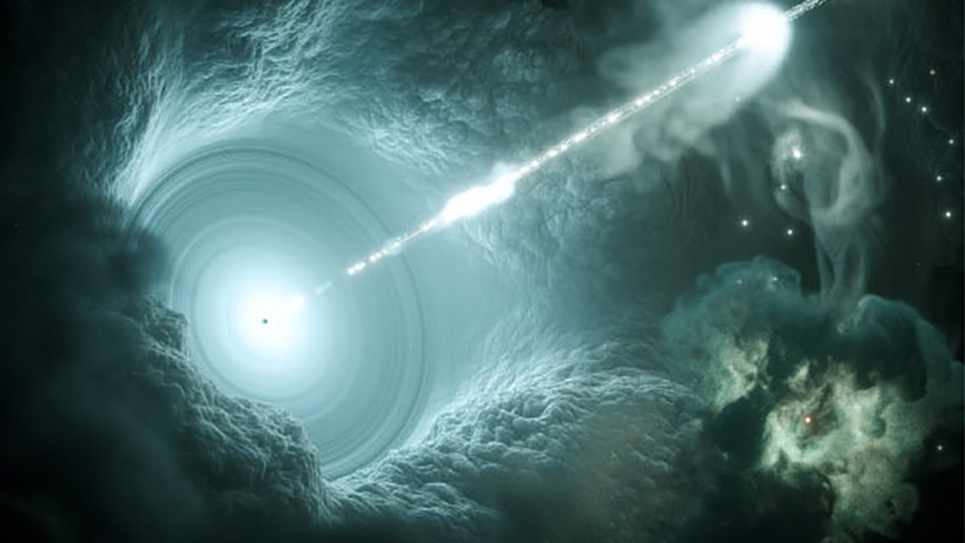
Machine Learning and Genetic Algorithms in Theoretical Physics
When constructing mathematical models of fundamental physics, one often encounters large parameter spaces that defy systematic scans. In the first part of this talk I will give a quick overview of particle physics and string theory model building and the origin of the large landscapes of theories one encounters. Then I will explain how computational methods, specifically reinforcement learning and genetic algorithms, can be deployed successfully to explore these landscapes and identify models with prescribed properties. In particular, I will show how previously unknown string standard models can be found in this way.
Bio:
I am a second year DPhil student, in the Particle Theory subdepartment of the Rudolf Peierls Centre for Theoretical Physics, working under the supervision of Prof Andre Lukas. Before coming to Oxford, I completed an MSci in Theoretical Physics at Royal Holloway in 2019 and Part III of the Mathematical Tripos at Cambridge in 2020. While at Royal Holloway I undertook a research review on black hold physics, and a major project on unitarity inspired methods for loop integrals.
Location: Click here to join via MS Teams