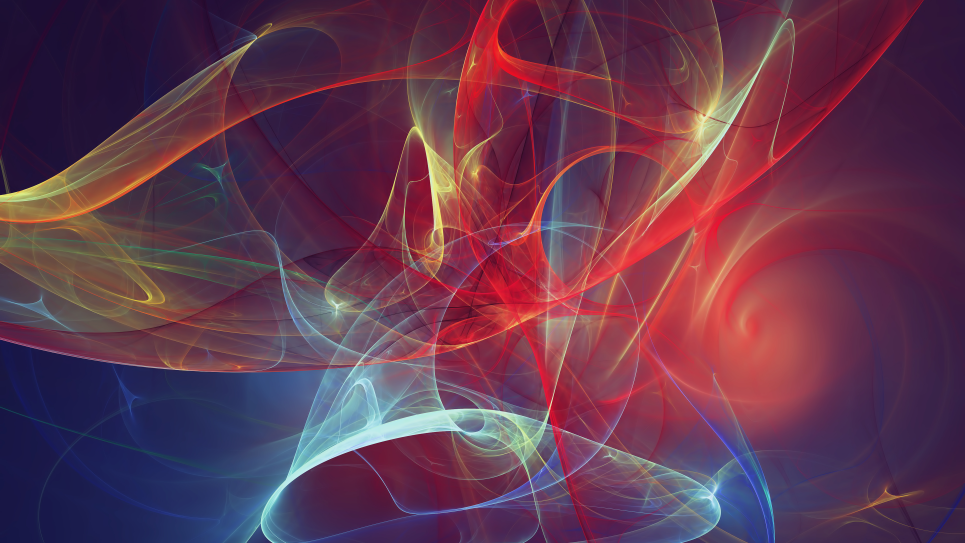
Existing regularization methods of the ensemble Kalman filter (EnKF) assume that the observations are local functions of the state. We present a regularization of the EnKF for non-local observations such as solutions of elliptic partial differential equations. Elliptic inverse problems are usually highly compressible: low dimensional projections of the observations strongly inform a low-dimensional subspace of the state space. To leverage this structure, we derive a low-rank factorization of the Kalman gain based on the spectrum of the Jacobian of the observation operator.
From the rapid spectral decay, the inference can be performed in the low-dimensional subspace spanned by the dominant eigenvectors. However, the low-rank EnKF (LREnKF) remains biased for nonlinear state-space models. To reduce this bias, we develop a nonlinear generalization of the LREnKF, where the low-dimensional linear transformation is replaced by a sparse and interpretable low-dimensional nonlinear transformation based on measure transport. These low-rank filters are assessed on potential flow problems.