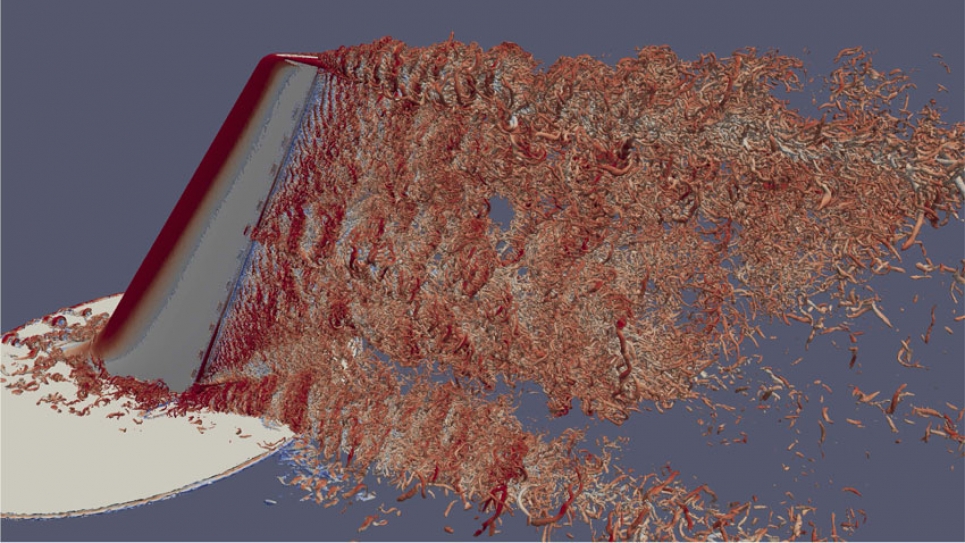
Large eddy simulations (LES) are gaining popularity with increased access to vast computational resources and increasingly used for high-fidelity simulations of flows for which DNS is still out of reach. LES involves solving filtered Navier-Stokes equations that are unclosed due to the presence of subgrid stress (SGS) tensor accounting for the interaction between unresolved and resolved turbulent scales. Several SGS models are available in the community, however, they are inadequate for high Reynolds number wall-bounded flows. Therefore, data-driven models are being considered lately as an approach to address this issue.
In this presentation, we propose a data-driven model for the SGS tensor that leverages high-fidelity open-source DNS data. Appropriate selection of model inputs and outputs and their representation in a flow-based eigen-frame enables the trained model to satisfy physical invariance conditions such as Galilean, time, unit, and frame invariance without any data augmentation. The methodology is further extended to anisotropic grids. The selected inputs and outputs allow us to train the model using a simple neural network with a relatively small amount of data, thereby reducing the model evaluation cost. We conduct A priori and A posteriori tests with the trained model and demonstrate its applicability for Reynolds numbers and flow physics outside the training dataset.
Zoom Link: https://argonne.zoomgov.com/j/1601852284?pwd=TW1LOWYzM0FMVTFtRkxkbHM5ZElJQT09