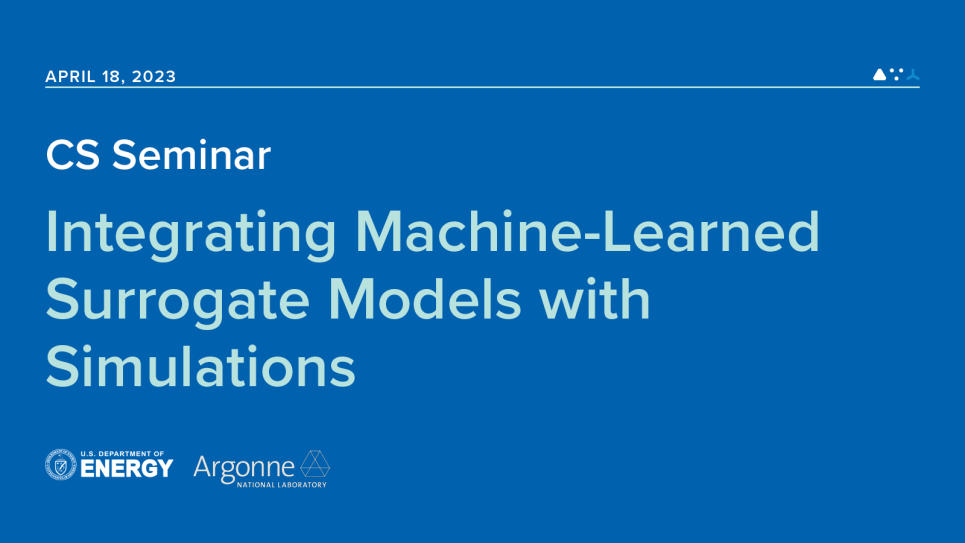
Simulations can be computationally expensive, so it can be advantageous to use machine learning to train a surrogate model that is orders of magnitude faster. However, completely data-driven black-box models often have disadvantages such as limited generalizability and the chance of physically-impossible predictions. I will describe our recent work on surrogate modeling for applications such as automotive engines and weather, as well as how we are creating hybrid models by integrating surrogate models back into simulations. I will include some ongoing computer science challenges in integrating machine learning with simulations.
Bethany Lusch
Dr. Bethany Lusch is an Assistant Computer Scientist in the Data Science group with the Argonne Leadership Computing Facility (ALCF) at Argonne National Laboratory. Her research expertise includes developing methods and tools to integrate AI with science, especially for dynamical systems and PDE-based simulations. Her recent work includes developing machine-learning emulators to replace expensive parts of simulations, such as computational fluid dynamics simulations of engines and climate simulations. She is also working on methods that incorporate domain knowledge in machine learning, representation learning, and using machine learning to analyze supercomputer logs. She holds a PhD and MS in applied mathematics from the University of Washington and a BS in mathematics from the University of Notre Dame.