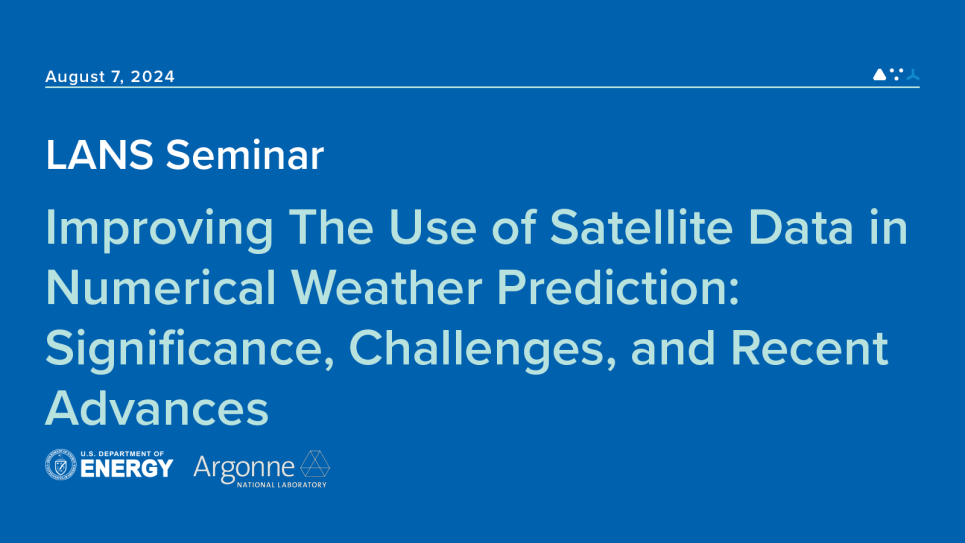
Data assimilation systems (DAS) for numerical weather prediction (NWP) combine information from a numerical model, observational data, and error statistics to analyze and predict the state of the atmosphere. Four-dimensional variational methods (4D-Var) produce an estimate (analysis) to the true state by solving a large-scale model-constrained optimization problem. The rapid growth in the data volume provided by satellite-based instruments has prompted research to assess and improve the forecast impact (''value'') of high-resolution observations. This talk presents theoretical and practical aspects of hyperparameter sensitivity analysis in a 4D-Var DAS including evaluation of the forecast sensitivity to observations (FSO), prior state estimate, and parameterized error covariance models. An FSO-based optimization approach is formulated to identify deficiencies in the weight assigned to various observing system components and adaptively improve the use of observations. The practical ability to implement this methodology is demonstrated in a computational environment that features all elements necessary for applications to NWP.
Bio: Dacian Daescu, is a Professor of Fariboz Meseeh Mathematics and Statistices in the Liberal Arts & Sciences Department of Portland State University. Prof. Daescu research interests are in applied and computational mathematics and data-enabled science to analyze and predict the state of complex dynamical systems that are mathematically modeled by nonlinear ordinary differential equations (ODEs) or partial differential equations (PDEs) systems. His application-oriented research on data assimilation, model-constrained optimization, sensitivity analysis, and uncertainty quantification relies on state-of-the-art numerical optimization algorithms, high-performance computing, inverse problems theory and statistical data analysis tools to improve the models' predictive capabilities and to develop new methodologies for observing system assessment, experimental design, and accurate representation of the uncertainty in model forecasts. Specific applications include geophysical and environmental problems of major societal impact such as numerical weather prediction and air pollution forecasting. Prof. Daescu’s research at PSU has been supported by grants and awards from NASA, NRL, NSF, and Intel Inc.
See all upcoming talks at https://www.anl.gov/mcs/lans-seminars