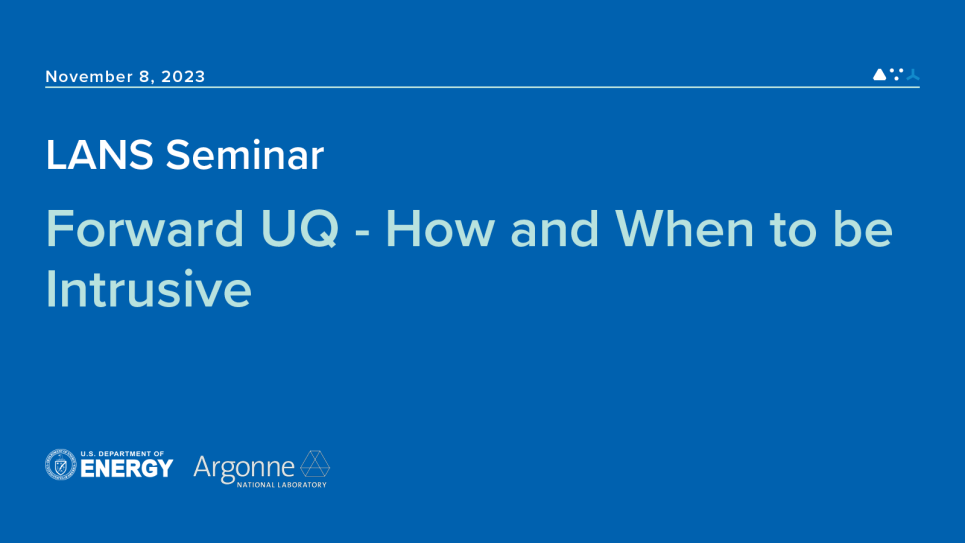
In engineering applications (heat transfer, fluid flow, elasticity etc), we often encounter physics-based models consisting of partial differential equations (PDEs) with uncertain inputs, which are reformulated as parametric PDEs. In the field of Uncertainty Quantification (UQ), we typically model uncertain inputs as random variables. Given a probability distribution for the inputs, the forward UQ problem then consists of trying to estimate statistical quantities of interest related to the model solution. Over the last two decades, many numerical schemes have been developed to tackle both forward and inverse problems involving PDEs. The vast majority are sampling schemes and are non-intrusive in the sense that users do not have to modify existing solvers for the associated deterministic PDEs. This is very attractive in industrial settings. Stochastic Galerkin methods, also known as intrusive polynomial chaos methods, standard apart and are much less widely used in practice. Key challenges include: how to design the Galerkin approximation space, and how to solve the associated linear systems of equations. In this talk, I will outline the intrusive approach for forward UQ in PDE models, advantages and limitations, and emphasise the importance of adaptivity.
Bio: Catherine Powell is a Professor of Applied Mathematics at the University of Manchester, UK. She is a numerical analyst whose research centres on aspects of approximation theory, error estimation and linear algebra for discretisations of partial differential equations (PDEs) and PDEs with random or parameter-dependent inputs. She is currently serving as President of the SIAM UK and Ireland Section and is associate editor for the SIAM Journal on Numerical Analysis and the SIAM/ASA Journal on Uncertainty Quantification.
See all upcoming talks at https://www.anl.gov/mcs/lans-seminars