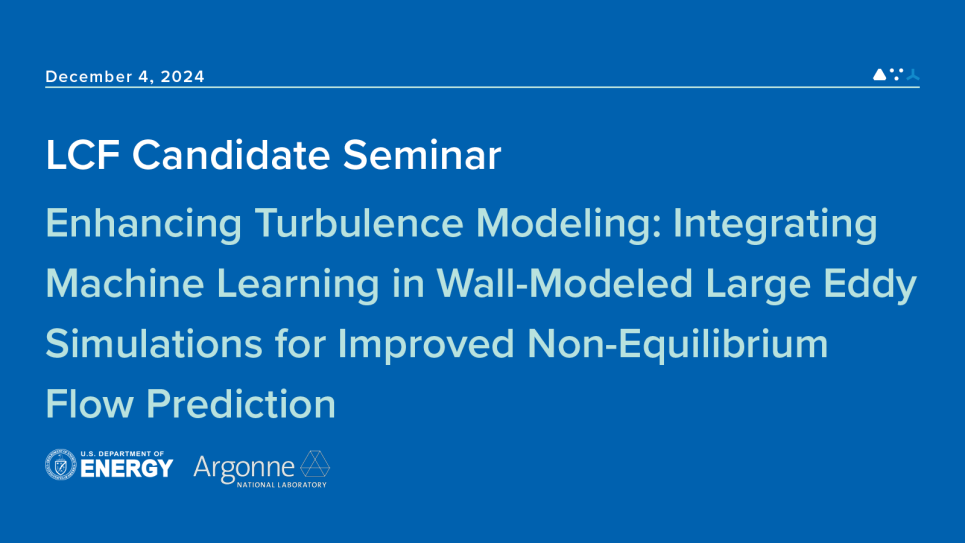
Wall-modeled large eddy simulation (WMLES) oFers a balance between accuracy and computational feasibility in turbulence modeling, especially in high-Reynolds-number flows. While WMLES reduces computational costs relative to fully resolved LES, wall models face challenges under non-equilibrium conditions, such as pressure gradients, curvature, and separation. My doctoral research has focused on developing a novel sensorbased wall modelling approach to improve relaminarization predictions driven by pressure gradients, validated on the flow over a Gaussian-shaped bump geometry. This seminar will cover key findings from this work and outline my proposed research to integrate machine learning for a more accurate, data-driven wall model. This approach promises to enhance WMLES’s predictive accuracy for complex flows considering non-equilibrium eFects on leadership-class computing systems, with the potential to advance predictive capabilities in turbulence modeling.