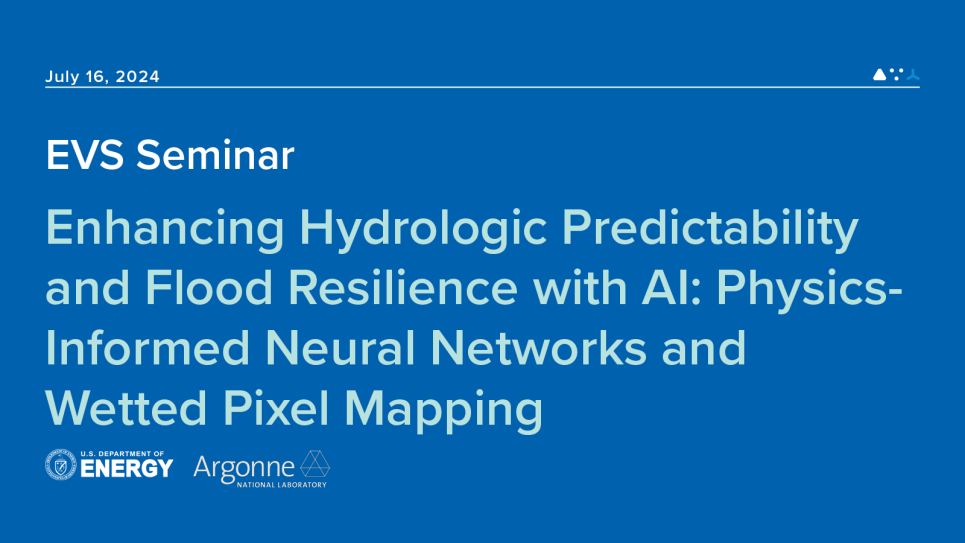
The growing risk of extreme precipitation has underscored the need for high resolution, accurate flood maps. Traditional numerical methods for flood modeling face significant challenges, including intense computational burdens and model misspecification due to missing hydrologic modeling mechanisms. These challenges create an opportunity to employ novel artificial intelligence (AI) solutions that can speed up flood simulations and improve prediction accuracy using observation records. This seminar presents two ongoing research tasks that aim to enhance the predictability of hydrologic systems using AI: (1) A computer vision AI model that generates flood observation records from multispectral and synthetic aperture radar data, and (2) Physics-based neural networks that serve as surrogate models for hydrodynamic processes. Both projects leverage novel training techniques and demonstrate the important role of deep learning in improving hydrologic systems predictability. This research will ultimately be used to address the problems of computational burden and model misspecification by speeding up CPU-bound processes and improving prediction accuracy with flood observations following extreme precipitation events, enabling greater flood resilience.