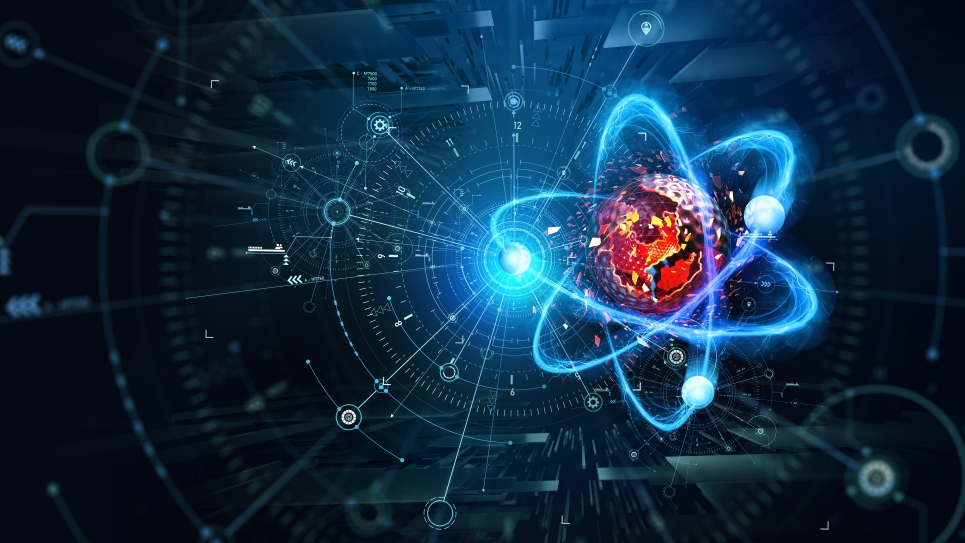
Understanding the physical phenomena in nature, typically governed by differential equations, is fundamental to the science and engineering disciplines. Hence, huge research efforts have been devoted to data-driven approaches for scientific problems thanks to the explosive measurement data and the advancement of machine learning algorithms. However, the obstacles still exist due to the generalization, interpretability and scalability issues. To this end, we propose the novel physics-informed/encoded deep learning frameworks for forward and inverse analysis of spatiotemporal systems.
Specifically, we present our transitions in two branches: (i) from continuous learning to discrete learning; (ii) from physics-informed learning to physics-encoded learning. Through comprehensive numerical experiments, we demonstrate the effectiveness and generalizability of our proposed methods in solving complex PDEs, scientific data augmentation, and inverse discovery of governing equations from scarce measurement data.