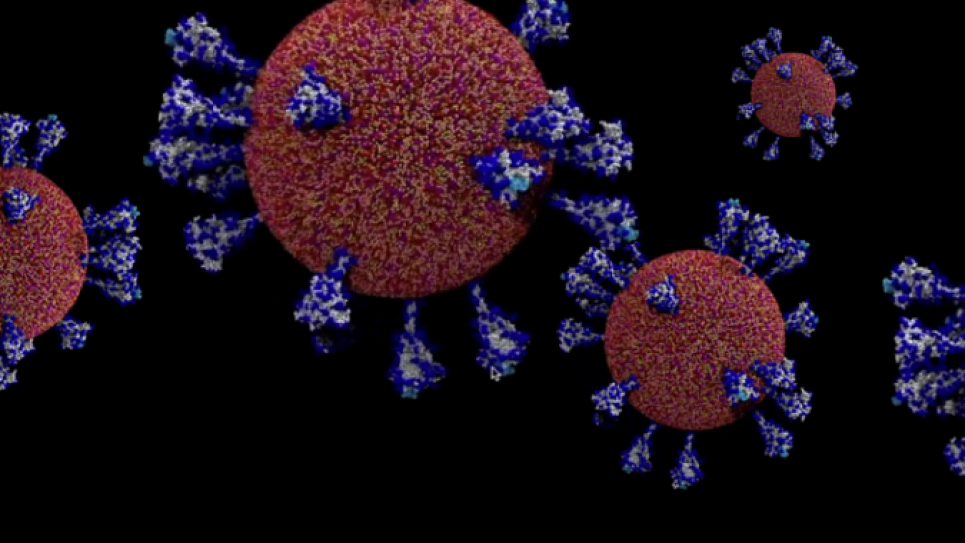
Abstract: The SARS-CoV-2 Delta variant (B.1.617.2) was initially identified in India in December 2020. Due to its high transmissibility, its prevalence in the USA grew from a near-zero baseline in early May 2021 to nearly 100% by late August 2021. The question of whether Delta is or is not a vaccine "escape" variant is of urgent importance to public health authorities, and has been debated, somewhat inconclusively, in the scientific literature. We gathered openly available data sources on RT-PCR COVID-19 tests split by the COVID-19 vaccination status of individuals tested during this period from the public health authorities of seven U.S. states, five U.S. counties, and the District of Columbia. This data enabled us to generate geographically-resolved time-series of vaccine effectiveness (VE) against test-diagnosed infection, concurrently with the growth of Delta variant prevalence in those locations. Our analyses reveal that in each locality the VE for the combined set of all three US vaccines remained relatively stable and quite well-performing, despite the dramatic concurrent rise of Delta variant prevalence. We conclude that the Delta variant is not a vaccine escape variant to any significant degree. We discuss further implications of this type of data for epidemic surveillance, including opportunities for simple data reforms and expansion of COVID-19 test data collection, curation, and distribution in other public health authorities in the USA and Internationally. Such reforms could result in the creation of a real-time COVID-19 epidemic observatory with surveillance capabilities that exceed anything available at present.
Bio: Carlo Graziani is a computational scientist at Argonne National Laboratory. He received a PhD in physics from the University of Chicago in 1993. He works on problems in probabilistic machine learning,
Bayesian statistics, and uncertainty quantification in computational science applications.
Location: https://uchicagomedicine.zoom.us/j/95142867968?pwd=dng1Q0JOanFvRzdIeURVSkJBQmhvUT09