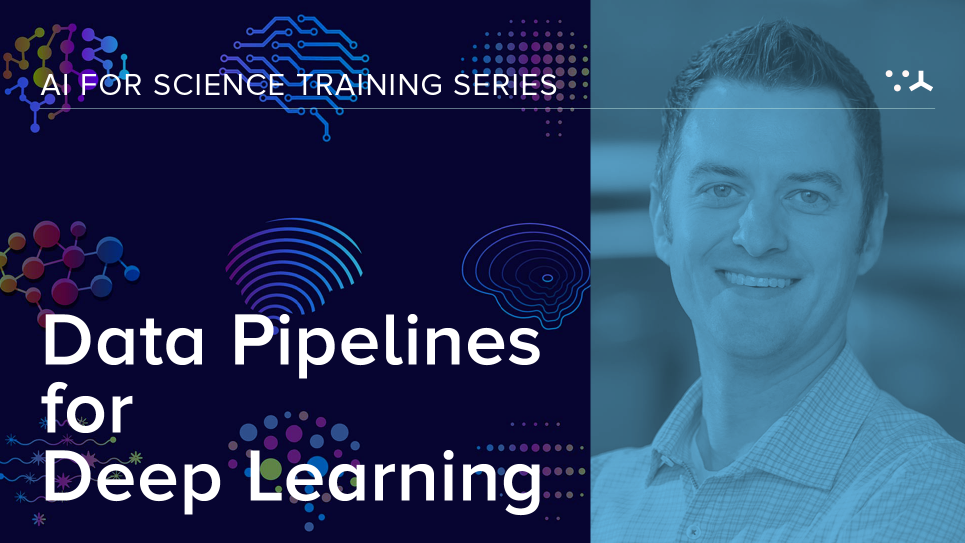
This module will equip students to adequately handle datasets and AI models in the ThetaGPU supercomputer for AI research.
Day and Time: December 2, 3-5 p.m. US CT
This session is a part of the ALCF AI for Science Training Series.
Taylor Childers has a PhD in Physics from Univ. of Minnesota. He worked at the CERN laboratory in Geneva, Switzerland for six years as a member of the ATLAS experiment and a co-author of the Higgs Boson discovery paper in July 2012. He has worked in physics analysis, workflows, and simulation from scaling on DOE supercomputers to fast custom electronics (ASIC/FPGA). He applies deep learning to science domain problems including the use of Graph Neural Networks to perform semantic segmentation to associate each the 100 millions pixels of the ATLAS detector to particles originating from the proton collisions. He is currently working with scientists from different domains to apply deep learning to their datasets and take advantage of Exascale supercomputers that will be arriving in the next few years.