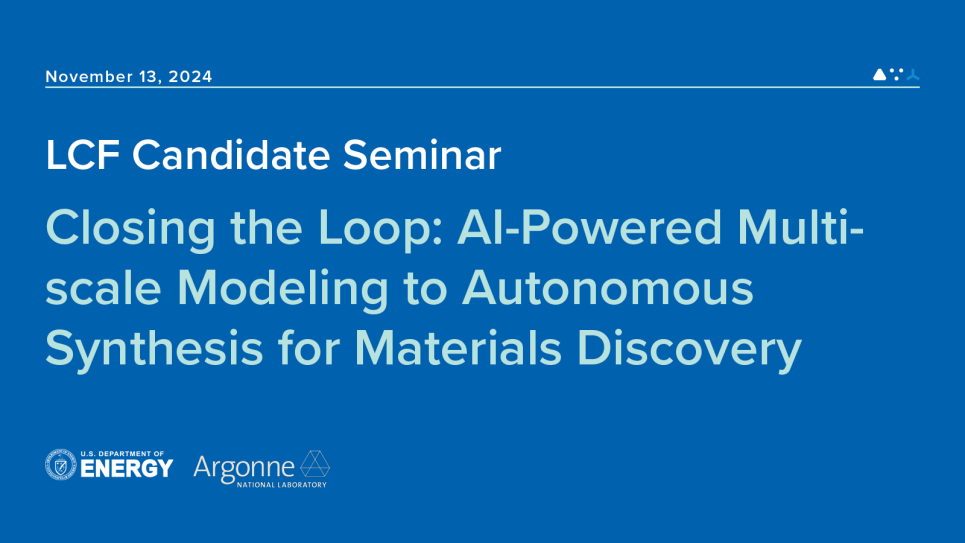
Multiscale modeling is essential for understanding materials across different scales and requires advanced methods to connect electronic, atomistic, and mesoscale descriptions. In this presentation, I will present a multi-fidelity approach using reinforcement learning (RL) to bridge these scales, focusing on silica. Its structural complexity and polymorphic diversity pose significant challenges for accurate property prediction, making it an ideal material system for validating transferable workflows. I will also discuss autonomous parameter tuning through meta-learning and how developed models can create intelligent surrogates for autonomous synthesis. Additionally, I will cover foundational models derived from complex graph-neural networks and interpretable functional forms obtained from datasets using symbolic regression. Finally, I will propose strategies to leverage the extensive datasets generated during model development and application, in conjunction with experimental data sources, through a foundational knowledge graph aimed at achieving autonomous materials discovery.