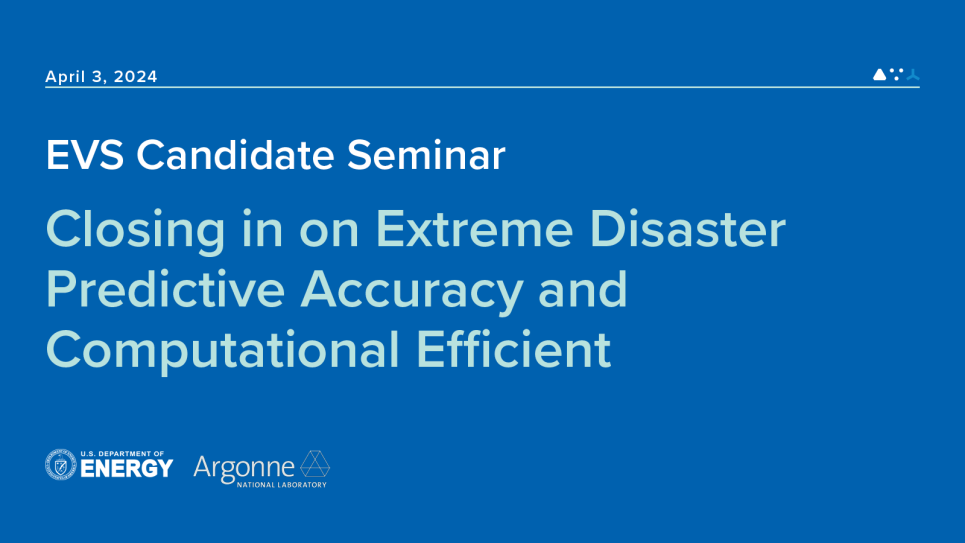
Flooding is becoming increasingly severe in urban areas, due to an increase in heavy rainfall coupled with a high concentration of impervious surfaces, which causes flooding outside of river and floodplain areas (known as ‘pluvial-urban’ flooding). This kind of flooding has caused catastrophic damage and loss of life across the nation, yet current forecasting is hampered by issues with accuracy, as it is impacted by uncertain sources and intensive computational costs when using high-fidelity models. In this talk, I will present a modeling framework that can tackle both of these problems by utilizing the power of sophisticated urban flood modeling (UFM), probabilistic “surrogate” modeling, and machine learning. The UFM combines surface drainage network and flow hydrodynamic features within an urban environment to forecast floods outside of riverine areas. The flood “surrogate” combined with high-fidelity modeling is essential for constructing practical tools to boost urban resilience to pluvial flooding in real time. The strength of machine learning can be employed to quantify unknown aleatoric and epistemic uncertainties that influence modeling results. This research will provide vital knowledge on urban flooding, allowing us to quantify the probability of a flood hazard outside of riverine floodplains, to aid disaster management planning on an engineering scale.