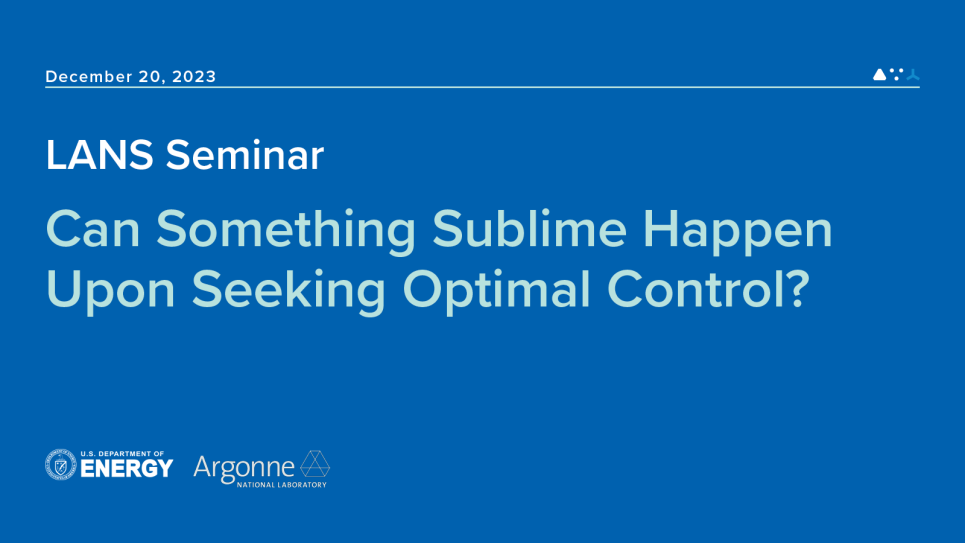
Optimal control is pervasive with the nature of the objectives being vast along with the controls involved being diverse. A common ground for all optimal control problems is that they have a landscape which is the objective as a function of the controls. The topology of such landscapes greatly influences the ease of obtaining maximum control performance. The presentation will consider control in various areas of science, including quantum dynamics, chemical and material science, natural evolution, and directed evolution. Controlled quantum dynamics phenomena have received considerable attention, as the associated landscape topology can be analyzed upon satisfaction of particular assumptions. This work was also extended to the control of a rather general class of coupled nonlinear ordinary differential equations, thereby opening up the scope of the formal landscape analysis. Importantly, quantum control experiments have verified the theoretically determined landscape topology in that domain. In the other areas of science mentioned above, while reliable models often don't exist, these domains are replete with experimental studies that either directly examine the associated control landscapes or implicitly draw conclusions on their nature. In chemical and material science, and directed evolution, the use of machine learning (ML) and like algorithms have been quite successful, which suggests that their control landscapes are rather smooth. The overall sublime finding is that in all of the listed domains of science, optimization is surprisingly easy (i.e., putting aside any overhead) despite the general exponentially large spaces of controls. Nature perhaps can be singled out to have first discovered the power of overparameterization with even ~ 4109 genomic controls. There is much interest in the landscape of artificial neural networks, especially in deep learning. An item of speculation is whether the
principles of control landscape theory might be applicable to ML parameter landscapes arising from big data.
Speaker Bio: Herschel Rabitz graduated from Harvard University in 1970, earning his Ph.D. in chemical physics. This was followed by post-doctoral work at the University of Wisconsin. In 1971 Professor Rabitz joined the faculty of the Princeton University Department of Chemistry, and from July 1993 to July 1996 he was chair of the department. He is also an affiliated member of Princeton University’s Program in Applied and Computational Mathematics. Professor Rabitz’s research interests lie at the interface of chemistry, physics, and engineering, with principal areas of focus including: molecular dynamics, biophysical chemistry, chemical kinetics, and optical interactions with matter. An overriding theme throughout his research is the emphasis on molecular scale.
See all upcoming talks at https://www.anl.gov/mcs/lans-seminars