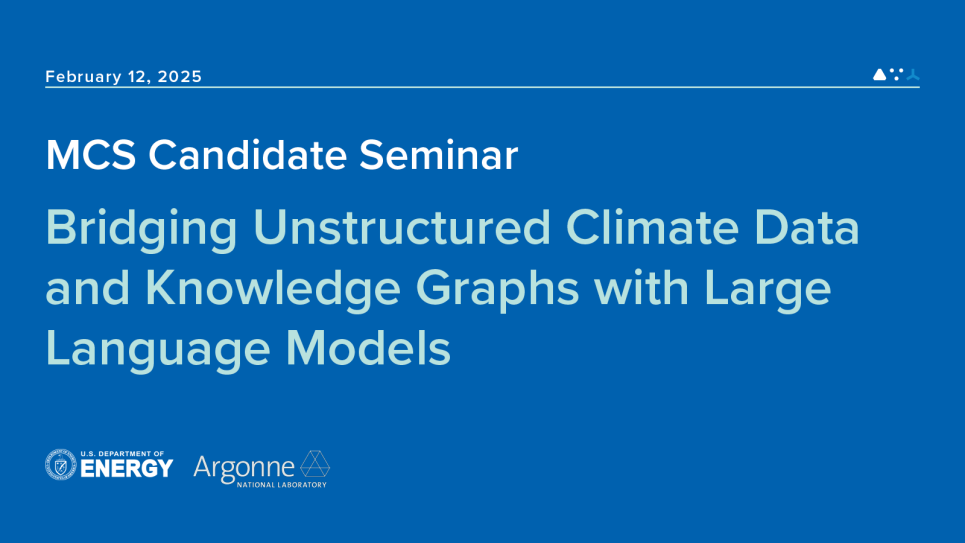
The growing volume of climate science literature presents challenges in extracting and organizing domain-specific knowledge. To address this, we propose a taxonomy-driven approach to constructing a climate science knowledge graph (KG) using NASA’s GCMD taxonomy as the backbone. Our method integrates Large Language Models (LLMs) enhanced with Retrieval-Augmented Generation (RAG) and few-shot learning to extract entities, link them to GCMD, and identify relationships such as “MeasuredAt” and RunBy” A three-stage annotation pipeline ensures high-quality outputs, validated by domain experts. We evaluate the approach with ablation studies and comparisons to alternative models, including OpenAI’s GPT and DeepSeek, highlighting the trade-offs between accuracy and computational cost. This work demonstrates the potential of LLMs to bridge the gap between unstructured climate data and structured representations, enabling advanced applications in climate research and decision-making.