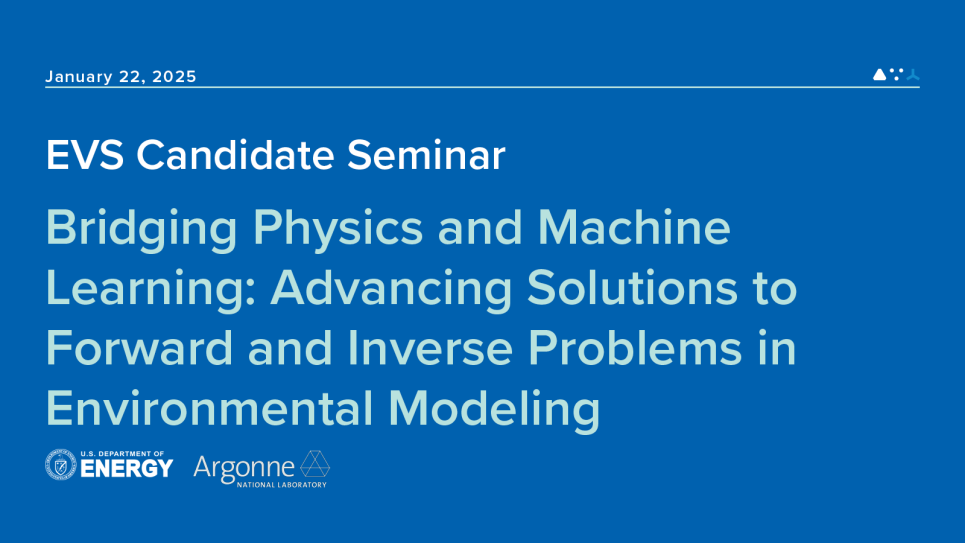
The integration of physics-based principles with machine learning techniques provides a robust framework for addressing forward and inverse problems in complex environmental systems. This seminar will present my research contributions toward developing hybrid methodologies that enhance predictive modeling and parameter estimation in domains such as oceanic and hydrology. Key advancements include the application of physics-informed neural networks to forward problems, such as forecasting wave dynamics, and the development of transformer-based models for inverse problems, including multi-location parameter inference. I will also discuss strategies to improve model interpretability, generalizability, and computational efficiency, emphasizing the role of data assimilation and physics-regularized learning. By leveraging these approaches, my work bridges domain-specific knowledge with state-of-the-art machine learning techniques, offering reliable and scalable solutions to critical scientific and environmental challenges. This research underscores the potential of hybrid methodologies to address complex systems and drive innovation in predictive modeling.