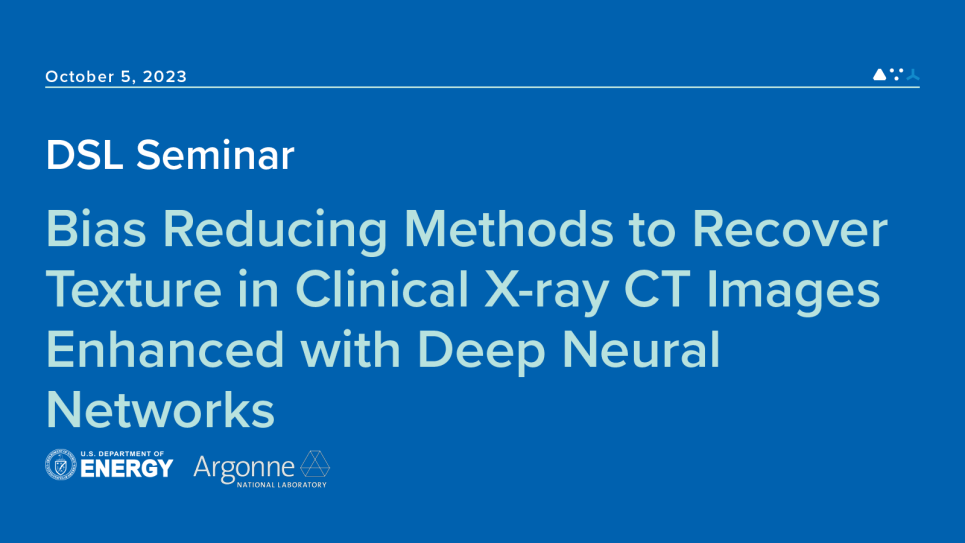
There is growing interest to use deep neural network (DNN) based denoising and sharpening for medical computed tomography (CT) images. An effective quality enhancement algorithm must retain a favorable texture since radiologists are confident with images containing traditional FBP (Filtered BackProjection)-like texture. However, commonly used DNN loss functions produce over-smooth (biased) images lacking texture.
This talk presents two algorithms to recover texture. The first method proposes a bias-reducing loss function that penalizes bias in the image more while training a DNN, producing more texture and detail in results. The amount of texture retained is controlled by a user-adjustable parameter. The second method, texture matching generative adversarial network (TMGAN), provides further flexibility to generate texture matching with a target (FBP-like) one in the results. Importantly, TMGAN separates anatomical features from texture to alleviate the risk of hallucination. We demonstrate that the TMGAN achieves favorable texture quantitatively and qualitatively for clinical exams.
To add to calendar:
Click on: https://wordpress.cels.anl.gov/cels-seminars/
Enter your credentials.
Search for your seminar
Click “Add to calendar”