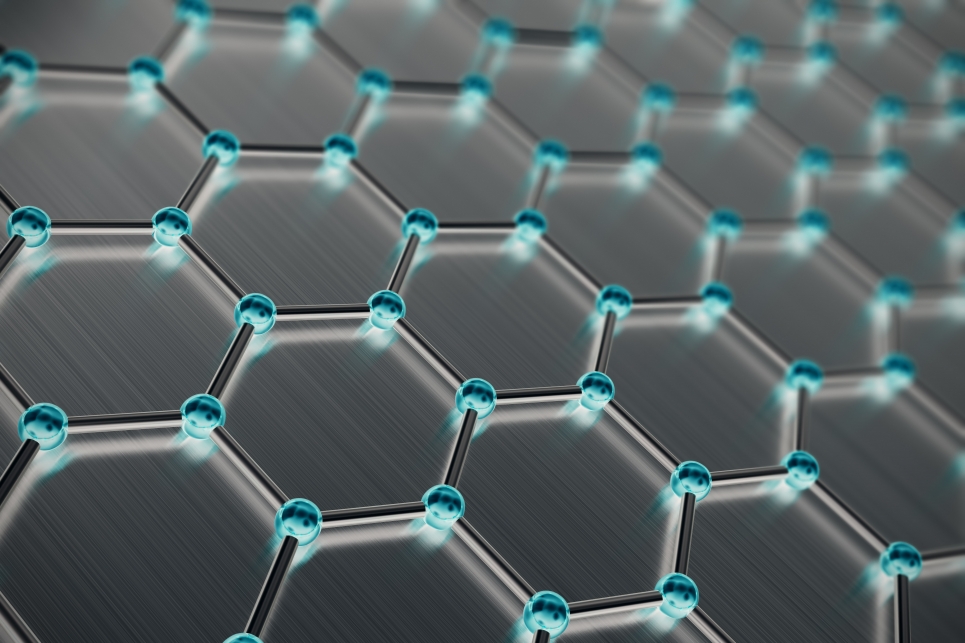
Shutterstock
Description: Finding strategies to synthesize novel functional materials and structures is one of the central endeavors of chemical and materials science. However, optimal material design involves a high dimensional parameter space search and time dependent sequential decision-making process, which results in development of new machine learning (ML) techniques that are capable of implicit decision-making over long period of time with little human supervision. In this talk, I will discuss our artificial intelligence driven approach based on offline reinforcement learning (RL) for automated discovery of quantum materials with targeted properties and design of strategies for their predictive synthesis. Further, I will discuss the mechanistic insight into material design and synthesis provided by our RL workflow in comparison to other ML techniques such as active learning and generative models. Finally, I will also discuss about recent work on designing graph neural network based interaction potential for molecular dynamics simulation and importance of inductive bias to learn such data driven potential energy surfaces in a sample efficient manner.