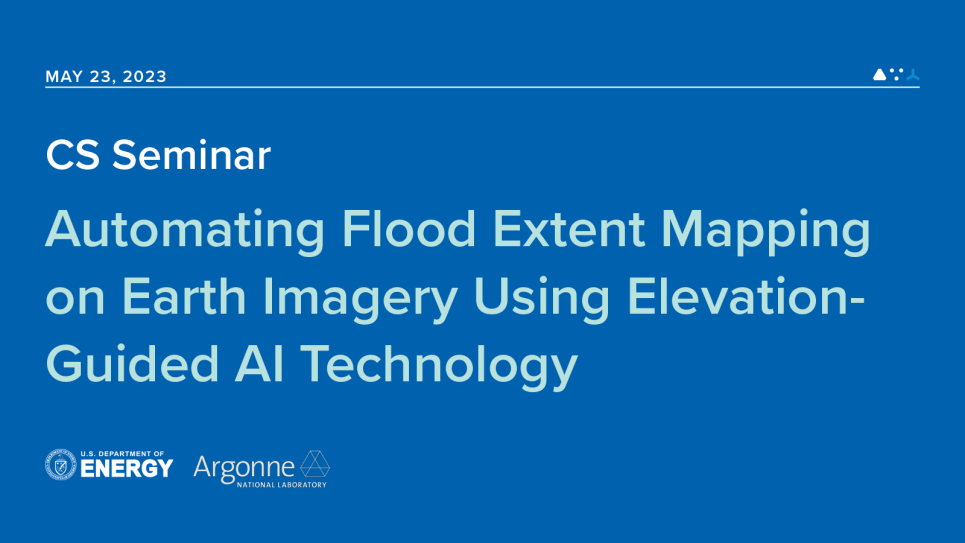
High-resolution optical imagery becomes increasingly available with the wide deployment of satellites and drones, and accurate and timely mapping of flood extent from the imagery plays a crucial role in disaster management such as damage assessment and relief activities. However, the problem is non-trivial due to the lack of ground truth flood maps, significant imagery noise and obstacles, complex spatial dependency on 3D terrains, spatial non-stationarity, and high computational cost. Existing machine learning approaches are mostly terrain-unaware and are prone to produce spurious results due to imagery noise and obstacles, requiring significant efforts in post-processing. To overcome this problem, we have developed an elevation-guided framework for accurate ground truth annotation, flood map prediction, and flood map refinement.
In this talk I will describe the 3 steps in our AI pipeline (1) Efficient annotation of disaster-time satellite images using 3D elevation-guided technology; (2) Elevation-guided flood map segmentation, with the model trained using the annotated images; (3) Elevation-guided flood map refinement using a graphical model called hidden Markov tree. Finally, I will talk about our ongoing works regarding Active Learning to speed up the annotation process and parallelization of our post-processing algorithm to speed up the computation.