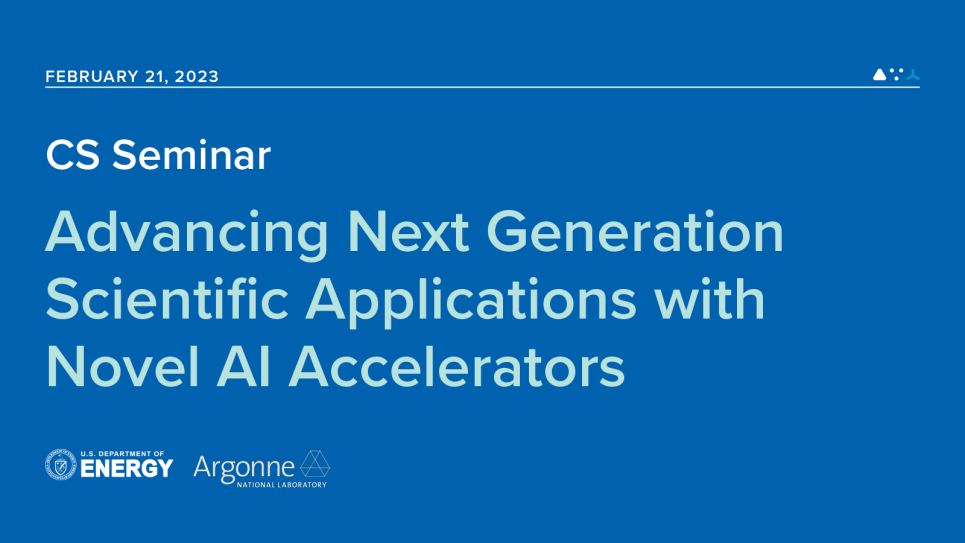
Scientific applications are increasingly adopting Artificial Intelligence (AI) techniques to advance science. High performance computing centers are evaluating emerging novel hardware accelerators to efficiently run AI-driven science applications. With a wide diversity in the hardware architectures and software stacks of these systems, it is challenging to understand how these accelerators perform. First, I will present an overview of the ALCF AI Testbed that houses novel AI accelerators from SambaNova, Cerebras, Graphcore, Groq, and Habana, with the goal to understand the efficiency and efficacy of these systems to accelerate AI for science applications. Next, I present a detailed evaluation of these accelerators with diverse workloads, such as Deep Learning (DL) primitives, benchmark models, and scientific machine learning applications along with performance of collective communications and scaling efficiencies. I will conclude with key insights, challenges, and opportunities in integrating these novel AI accelerators in supercomputing systems.
Speaker Bio:
Murali Emani is a computer scientist in the Data Science group with the Argonne Leadership Computing Facility (ALCF) at Argonne National Laboratory. His research interests are scalable machine learning, parallel programming models, high-performance computing, runtime systems, emerging HPC architectures, and online adaptation. Prior, he was a post-doctoral research staff member at Lawrence Livermore National Laboratory. He obtained his Ph.D. from the Institute for Computing Systems Architecture at the School of Informatics, University of Edinburgh. Murali published in top conferences, including PACT, PLDI, CGO, and SC, and has three granted patents. He was involved with the publication that won the ACM Gordon Bell prize for High-performance computing for Covid-19 at SC22. He served as a technical program committee member for conferences, including ISC’23, IPDPS’22, IPDPS’21, CCGRID’19, PACT’18, CCGRID’18, and ICPP’18. He is the co-founder and a co-chair for the MLPerf HPC group with MLCommons.