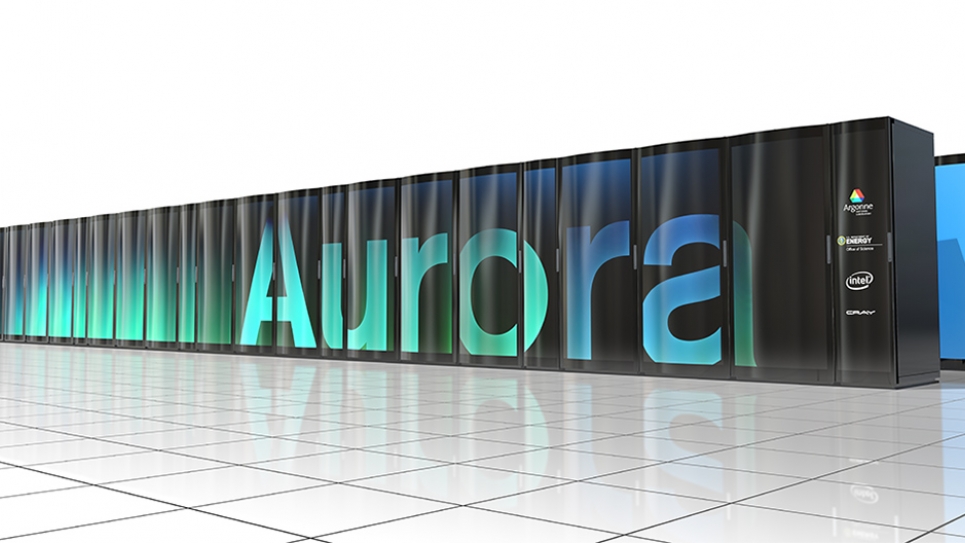
Science has always relied on a combination of approaches to derive an answer or develop a theory. The seeds for Darwin’s theory of natural selection grew under a Herculean aggregation of observation, data, and experiment. The more recent confirmation of gravitational waves by the Laser Interferometer Gravitational-Wave Observatory (LIGO) was a decades-long interplay of theory, experiment, and computation.
Certainly, this idea was not lost on the U.S. Department of Energy’s (DOE) Argonne National Laboratory, which has helped advance the boundaries of high-performance computing technologies through the Argonne Leadership Computing Facility (ALCF).
Realizing the promise of exascale computing, the ALCF is developing the framework by which to harness this immense computing power to an advanced combination of simulation, data analysis, and machine learning. This effort will undoubtedly reframe the way science is conducted, and do so on a global scale.
Since the ALCF was established in 2006, the methods used to collect, analyze and employ data have changed dramatically. Where data was once the product of and limited by physical observation and experiment, advances in feeds from scientific instrumentation such as beamlines, colliders, and space telescopes—just to name a few—have increased data output substantially, giving way to new terminologies, like “big data.”
While the scientific method remains intact and the human instinct to ask big questions still drives research, the way we respond to this new windfall of information requires a fundamental shift in how we use emerging computing technologies for analysis and discovery.
This convergence of simulation, data, and learning is driving an ever-more complex but logical feedback loop.
Increased computational capability supports larger scientific simulations that generate massive datasets used to feed a machine learning process, the output of which informs further and more precise simulation. This, too, is further augmented by data from observations, experiments, etc., to refine the process using data-driven approaches.
“While we have always had this tradition of running simulations, we’ve been working incrementally for more than a few years now to robustly integrate data and learning,” says Michael Papka, ALCF director and deputy associate laboratory director for Computing, Environment and Life Sciences (CELS).
To advance that objective, the facility launched its ALCF Data Science Program in 2016 to explore and improve computational methods that could better enable data-driven discoveries across scientific disciplines. The ALCF also recently expanded its Aurora Early Science Program with the addition of 10 new projects that will help prepare the facility’s future exascale supercomputer for data and learning approaches.
And earlier this year, the CELS directorate announced the creation of the Computational Science (CPS) and Data Science and Learning (DSL) divisions to explore challenging scientific problems through advanced modeling and simulation, and data analysis and other artificial intelligence methods, respectively.
“These combined efforts will focus on domain sciences and identify important problems that can be addressed through a combination of simulation, data science, and machine learning approaches. In many cases, we’ll be drawing on people with relevant expertise across multiple divisions,” says CPS director Paul Messina.
Already, this combination of programs and entities is being tested and proved through studies that cross the scientific spectrum, from understanding the origins of the universe to deciphering the neural connectivity of the brain.
Convergence for a brighter future
Data has always been a key driver in science and yes, it’s true that there is an exponentially larger amount than there was, say, ten years ago. But while the size and complexity of the data now available poses challenges, it is also providing opportunities for new insights.
No doubt Darwin’s research was big data for its time, but it was the culmination of nearly 30 years of painstaking collection and analysis. He might have whittled the process considerably had he had access to high-performance computers, and data analysis and machine learning techniques, such as data mining.
“These techniques don’t fundamentally change the scientific method, but they do change the scale or the velocity or the kind of complexity you can deal with,” notes Rick Stevens, CELS associate laboratory director and University of Chicago professor.
Take, for example, research into new materials designed to generate solar power as sunlight passes through windows. The technology has been hampered for lack of the right dye molecule, the discovery of which requires the time-consuming task of searching through reams of chemistry literature to find molecules with the right parameters.
Chemist and physicist Jacqueline Cole leads a collaborative effort between Argonne and the University of Cambridge to bring such molecules to light. Cole has developed a multi-stage process that cycles through simulation; data extraction, enrichment, and mining; materials prediction and experimental validation.
The team runs large-scale simulations on targeted molecules to predict chemical dyes with key optical properties. From these data, molecules are selected for synthesis, and the resulting chemicals are fabricated into devices for validating their prospects in solar-powered windows. The results determine whether further investigation is required.
“There’s a positive feedback loop inherent in this,” she says. “Even if the validation process doesn’t go well, it can still provide some helpful insights. We might learn, for example, that we need to refine the structure-function relationships of the molecules for a particular application or add a new type of data to the existing data.”
A large part of the effort was focused on constructing a database of desirable organic molecules, much of which was compiled by data mining some 300,000 published research articles. The research was spurred by the Materials Genome Initiative, a government initiative to take functional materials to market much faster than the decades it once took.
“The advantage of this process is to really take away the old manual curation of databases, which is lifetimes of work, and reduce it to a matter of a few months. Ultimately, a few days,” says Cole.
One machine to bind them all
Whether it’s the search for very specific dye molecules or understanding key flow physics to develop more efficient wind turbine blades, the merging and flourishing of simulation, data, and learning is only possible because of the exponential and deliberate development of high-performance computing and data delivery systems.
“Supercomputer architectures are being structured to make them more amenable to dealing with large amounts of data and facilitate learning, in addition to traditional simulations,” says Venkat Vishwanath, ALCF data sciences lead. “And we are fitting these machines with massive conduits that allow us to stream large amounts of data from the outside world, like the Large Hadron Collider at CERN and our own Advanced Photon Source (APS) and enable data-driven models.”
Many current architectures still require the transfer of data from computer to computer, from one machine, the sole function of which is simulation, to another that excels in data analysis and/or machine learning.
Within the last few years, Argonne and the ALCF have made a solid investment in high-performance computing that gets them closer to a fully integrated machine. The process accelerated in 2017, with the introduction of the Intel-Cray system, Theta, which is capable of combining traditional simulation runs and machine learning techniques.
The ALCF will help drive simulation, data, and learning to a new level in 2021, when they unveil the nation’s first exascale machine, Aurora. While it can perform a billion billion calculations per second, its main advantage may be its ability to conduct and converge simulation, data analysis, and machine learning under one hood. The end result will allow researchers to approach new types as well as much larger problems and reduce time to solution.
“Aurora will change the game,” says the ALCF’s Papka. “We’re working with vendors Intel and Cray to assure that we can support science through this confluence of simulation, data, and learning all on day one of Aurora’s deployment.”
Whether by Darwin or Turing, whether with chalkboard or graph paper, some of the world’s great scientific innovations were the product of one or several determined individuals who well understood the weight of applying balanced and varied approaches to support—or refute—a hypothesis.
Because current innovation is driven by collaboration among colleagues and between disciplines, the potential for discovery through the pragmatic application of new computational resources, coupled with unrestrained data flow, staggers the imagination.
The ALCF and APS are DOE Office of Science user facilities.
Argonne National Laboratory seeks solutions to pressing national problems in science and technology. The nation's first national laboratory, Argonne conducts leading-edge basic and applied scientific research in virtually every scientific discipline. Argonne researchers work closely with researchers from hundreds of companies, universities, and federal, state and municipal agencies to help them solve their specific problems, advance America's scientific leadership and prepare the nation for a better future. With employees from more than 60 nations, Argonne is managed by UChicago Argonne, LLC for the U.S. Department of Energy's Office of Science.
The U.S. Department of Energy's Office of Science is the single largest supporter of basic research in the physical sciences in the United States and is working to address some of the most pressing challenges of our time. For more information, visit the Office of Science website.