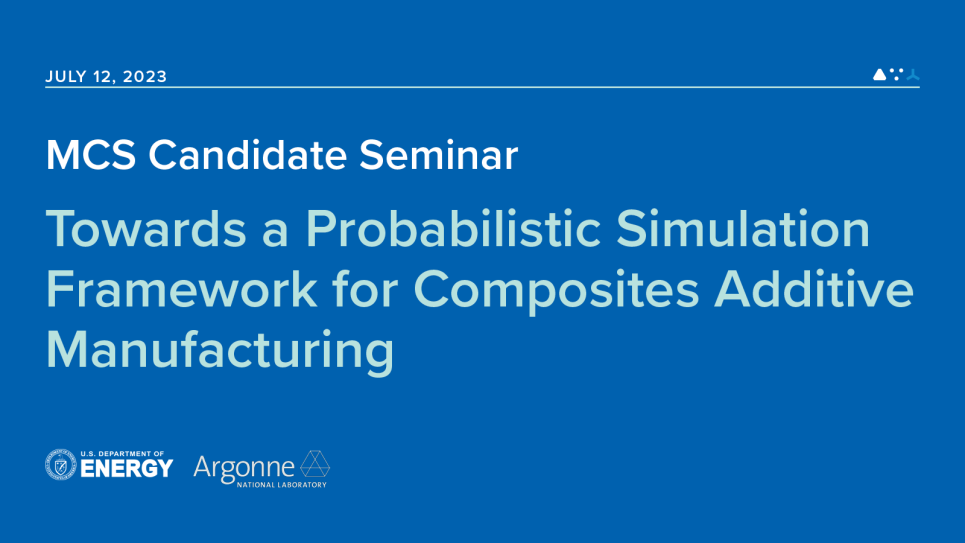
Developing composite manufacturing digital twins for short fiber-reinforced polymer composite processes, including injection molding and additive manufacturing requires extensive experimental material characterization. The first half of this research aims to address this experimental bottleneck by developing Bayesian methodologies to infer microstructural properties and, therefore enable a technology to transfer material properties across manufacturing systems. The developed method accounts for aleatoric and epistemic uncertainties, thereby make it a promising tool for a probabilistic predictive frameworks for composites manufacturing. However, to enable such frameworks, there is a need for parametric solutions of partial differential equations (PDEs) in composites manufacturing, since traditional solvers can be time consuming in high dimensional settings. The second half of the research addresses this computational bottleneck. A novel weak-form based formulation is developed to solve parametric PDEs enabled by neural networks. The method alleviates the need for estimating integrals, arising out of the weak form, using Gauss Quadrature and therefore reduces computational times significantly. The estimator developed is unbiased thereby supporting the convergence of stochastic gradient descent to train neural networks. The method is rigorously tested on parabolic and elliptical PDEs, and its extensions to parametric problems. The method is applied to solve layer wise addition of materials in composites manufacturing. This research makes fundamental contributions to computational science and lays the foundation for probabilistic predictive frameworks in composites additive manufacturing.