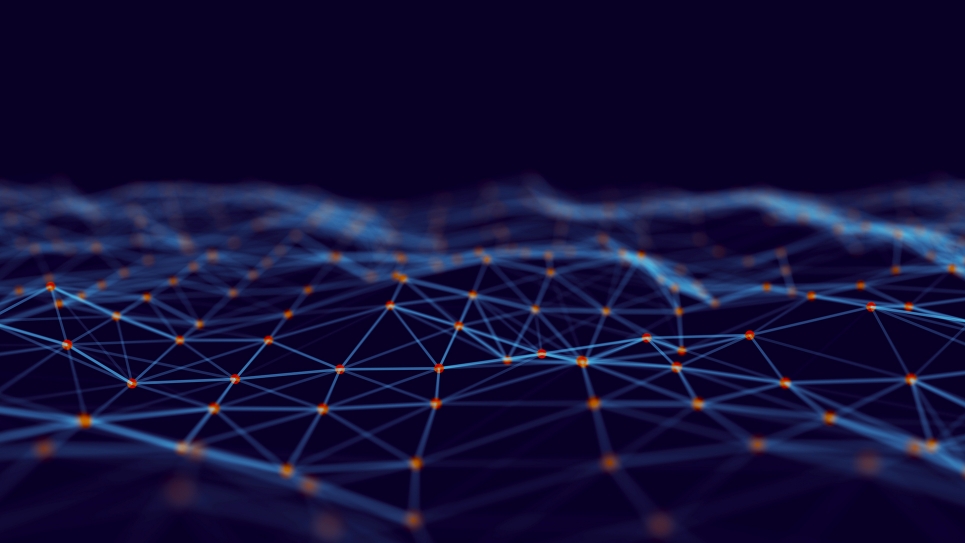
Data-driven models are stochastic due to the random distribution of the available data. Hence accounting for intrinsic and extrinsic errors in estimating the outcome of the models is crucial in optimizing them and rendering robustness in the optimization. We study the incorporation of model and data uncertainty in the general machine learning framework within the context of feature selection where we seek a subset of features that train models with the highest accuracy. The effect of the size and number of bootstraps in the underlying stochastic modeling and optimization are explored in real and synthetic datasets.
Please use this link to attend the virtual seminar: