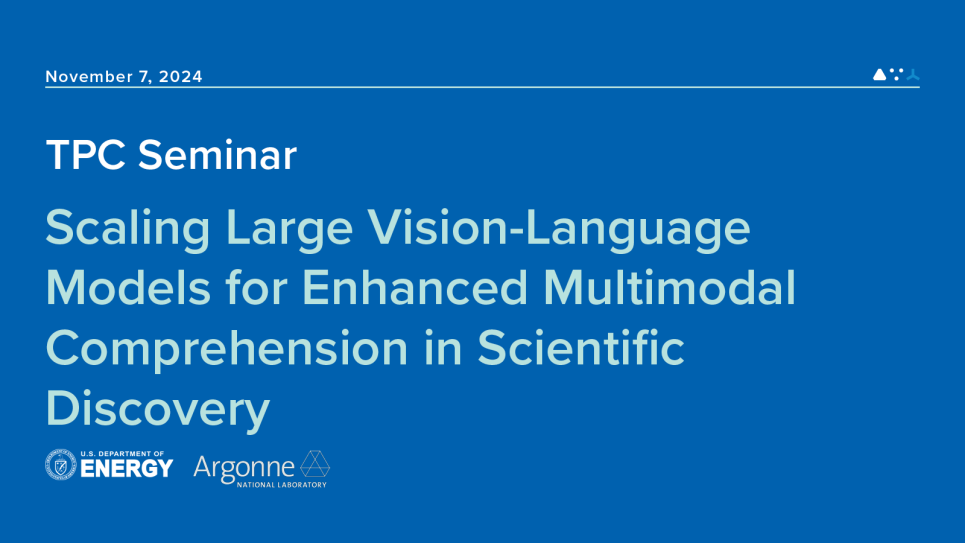
Chibuike Robinson Umeike is a fourth-year Ph.D. student in Civil, Construction, and Environmental Engineering at the University of Alabama, where he is also pursuing a Master’s degree in Computer Science. He is deeply committed to leveraging the concerted advantage of vision and language modalities for science and engineering applications. Robinson’s research has been featured in peer-reviewed IEEE and ASCE publications and presented at international conferences. Recently, he completed a research internship at Argonne National Laboratory's Data Science and Learning (DSL) division under the supervision of Neil Getty and Fangfang Xia, where he worked on developing scalable solutions for large vision-language models to accelerate scientific discovery and improve multimodal data comprehension.
Abstract: Large language models (LLMs) have demonstrated promising capabilities in understanding textual data and are increasingly being adopted to help researchers accelerate scientific discovery through knowledge extraction (information retrieval), knowledge distillation (summarizing key findings and methodologies into concise forms), and knowledge synthesis (aggregating information from multiple scientific sources to address complex queries, generate hypothesis and formulate experimental plans). However, scientific data often exists in both visual and textual modalities. Vision language models (VLMs) address this by incorporating a pretrained vision backbone for processing images and a cross-modal projector that adapts image tokens into the LLM dimensional space, thereby providing richer multimodal comprehension. Nevertheless, off-the-shelf VLMs show limited capabilities in handling domain-specific data. We propose two intelligent assistants finetuned from LLaVA, to help scale scientific discovery in low-dose radiation therapy (LDRT), a benign approach used in the treatment of cancer-related illnesses. Using multilingual data from 42673 scientific documents, we devise complex reasoning and detailed description tasks for visual question answering (VQA) benchmarks. Our preliminary experiments show that the scientific assistants, trained on 50,882 image-text data entries, outperform base LLaVA models by +1.8 points (52.1%) for v1.5-13b and +0.97 points (29.1%) for v1.6-vicuna-13b in overall performance, using Qwen2-72B-Instruct as the judge.