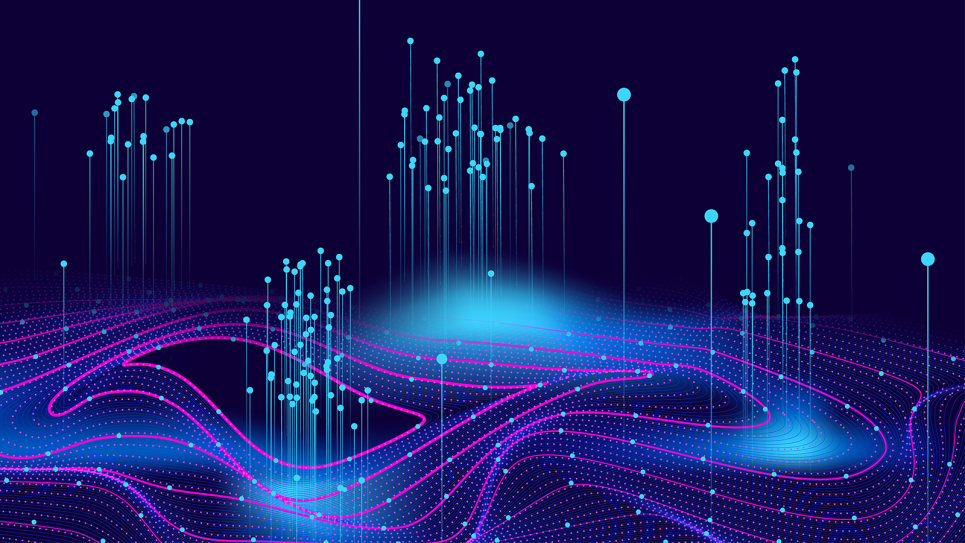
Multivariate Functional Approximation (MFA) is a technique for constructing an explicit functional representation of a data set for the purposes of analysis and visualization. MFA represents discrete data with a multidimensional piecewise-polynomial function (specifically, a B-spline) which can be used for analysis in place of the original data. Using a functional representation instead of interfacing with the data directly allows for derivatives, integrals, and point interpolations to be computed exactly, without resorting to additional approximations like finite differences or numerical quadrature. Therefore, the approximation error of these quantities is determined solely by the fit of the functional model to the original data, which eliminates the possibility of error propagating through several approximations. MFA's functional models can be iteratively refined to achieve a desired level of error, allowing users to select the level of precision needed by their application. This seminar will introduce MFA and its applications at a high level before delving into some of the mathematical challenges inherent in creating best-fit functional models with B-splines. Special attention will be devoted to the (potentially ill-conditioned) procedure for constructing best-fit B-spline models, as well as new techniques for improving the conditioning of this system. After describing basic MFA queries (evaluation, differentiation, integration), we will also discuss ongoing research into line integration with MFA.
Zoom Link: https://argonne.zoomgov.com/j/1600478193
See all upcoming talks at http://wordpress.cels.anl.gov/lans-seminars/